How Does Generative AI Work
Generative AI, powered by complex algorithms and machine learning, is revolutionizing various industries and changing the way we interact with technology. This cutting-edge technology has the ability to generate new content, such as images, music, and text, that is indistinguishable from human-created content. But how does generative AI actually work? Let’s dive into the details and explore the inner workings of this fascinating technology.
Key Takeaways:
- Generative AI uses complex algorithms and machine learning to create new content.
- This technology has applications in various industries, including art, music, and writing.
- Generative AI is based on models trained on vast amounts of data, enabling it to generate realistic content.
The Basics of Generative AI
At its core, generative AI works by analyzing massive amounts of data and using that information to create something new. It starts with a dataset that is used to train a generative model, such as a neural network. This model learns patterns and structures from the dataset, allowing it to generate content that follows similar patterns. *Generative AI is like an artist who learns from an artistic style and then creates new artworks inspired by that style.*
Once the generative model is trained, it can generate new content by taking in random input and mapping it to the patterns learned during training. For example, a generative model trained on images of dogs can generate realistic images of dogs when given random input. This process is often referred to as “sampling” or “inference”. *Through this process, generative AI can produce an infinite variety of creative outputs, each unique in its own way.*
Training Generative AI Models
Training generative AI models requires a large and diverse dataset. The more data available, the better the model can learn and generate high-quality content. Generative models are typically trained on datasets comprising thousands or even millions of examples. These datasets can include images, audio files, or text documents, depending on the type of content the generative model aims to create. *Training a generative AI model requires a significant amount of computational power and time, due to the complexity of the algorithms and the size of the dataset.*
During the training process, the generative model is fed input data and adjusts its internal parameters to minimize the difference between the generated content and the real content from the dataset. This optimization process is often performed using advanced techniques like gradient descent and backpropagation. *Through iterative training, the generative model gradually improves its ability to generate realistic and coherent content.*
Applications of Generative AI
Generative AI has a wide range of applications across different industries. It has been used to create stunning pieces of art, compose original music, and even write compelling stories or articles. The ability of generative AI to mimic human creativity opens up new possibilities for artists, musicians, and writers. *With generative AI, creators can collaborate with intelligent algorithms to push the boundaries of their own creativity.*
Industry | Application of Generative AI |
---|---|
Art | Generating unique and visually appealing artworks. |
Music | Creating original compositions and exploring new musical styles. |
Writing | Assisting authors in brainstorming ideas and generating storylines. |
Moreover, generative AI has found applications in industries such as fashion, design, and advertising. Companies are leveraging this technology to generate product designs, create realistic virtual prototypes, and even develop personalized marketing campaigns. *The possibilities of generative AI are vast and have the potential to transform various sectors in the coming years.*
The Future of Generative AI
As generative AI continues to advance, we can expect even more impressive creations and innovations. Improvements in computational power, as well as the availability of larger and richer datasets, will further enhance the capabilities of generative AI models. *Imagine a future where generative AI can create entire movies, design immersive virtual worlds, or compose symphonies indistinguishable from those of the greatest composers.*
However, with the increasing capabilities of generative AI, ethical considerations also come into play. Questions regarding intellectual property, authenticity, and control over AI-generated content will need to be addressed. *While generative AI offers immense opportunities, it also raises important societal and legal questions that must be carefully navigated.*
In Conclusion
Generative AI is a powerful technology that enables machines to create new and realistic content. By analyzing vast amounts of data and learning patterns, generative AI models can generate visually stunning artworks, compose original music, and even write compelling stories. *This technology has the potential to revolutionize creative industries and redefine the boundaries of human-machine collaboration.*
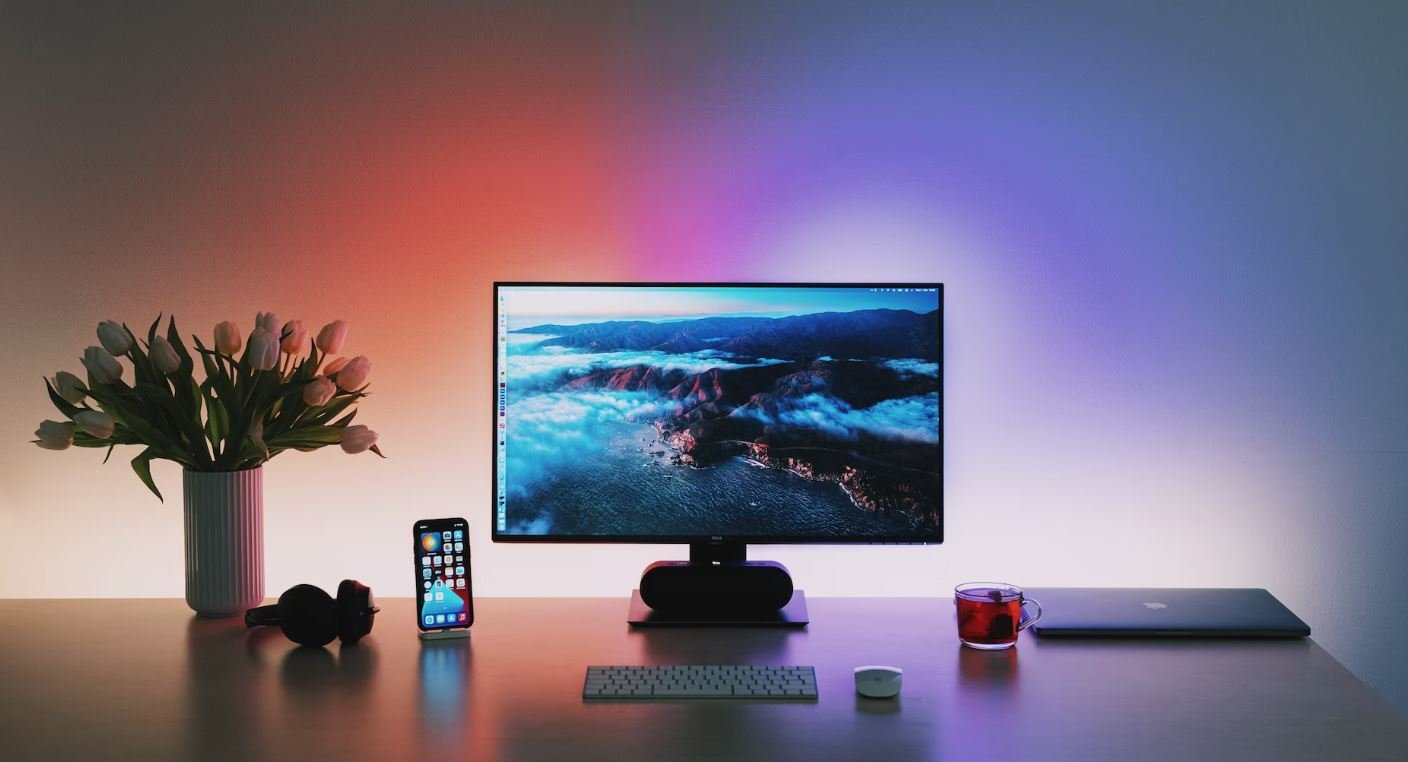
Common Misconceptions
Generative AI is the same as Artificial General Intelligence (AGI)
One common misconception people have is that Generative AI and Artificial General Intelligence (AGI) are the same thing. However, Generative AI refers to a specific type of AI technique that involves learning and generating new content, such as images, videos, or text. AGI, on the other hand, refers to a hypothetical AI system with general intelligence and capabilities equal to or surpassing human intelligence.
- Generative AI focuses on content generation
- AGI aims to replicate human intelligence
- Generative AI is a subset of AI technologies
Generative AI can replace human creativity
Another misconception is that Generative AI has the ability to replace human creativity entirely. While Generative AI can produce impressive pieces of content, it is still limited to what it has been trained on and lacks the nuance and intuition that humans possess. Generative AI is more commonly used as a tool to augment and inspire human creativity rather than replace it.
- Generative AI can assist artists and designers
- Human creativity is rooted in emotions and experiences
- Generative AI lacks originality and uniqueness
Generative AI can only create realistic content
Many people believe that Generative AI can only create content that is indistinguishable from reality. While Generative AI is capable of producing highly realistic content, it is not limited to that. Generative AI can also generate abstract and surreal compositions, expand on existing ideas, or even create content in a completely new and unique style.
- Generative AI can be used to create dreamlike art pieces
- Abstract content can be generated by tweaking AI models
- Generative AI can explore unconventional styles and perspectives
Generative AI is infallible and always produces perfect results
There is a misconception that Generative AI always produces perfect and error-free results. However, just like any other AI system, Generative AI is not flawless. It can still produce occasional artifacts, generate biased content, or make errors in output. Continuous research and development are necessary to improve the capabilities and mitigate these limitations.
- Quality of output can vary depending on training data and model
- Generative AI can sometimes exhibit bias present in the training data
- Post-processing may be required to refine and enhance generated content
Generative AI is easy to understand and control
Lastly, people often underestimate the complexity of Generative AI and assume it is easy to understand and control. However, the inner workings of Generative AI models can be highly complex, involving deep neural networks and intricate algorithms. Additionally, controlling and fine-tuning Generative AI systems requires expertise, careful consideration, and an understanding of the underlying technology.
- Generative AI involves complex mathematical computations
- Training AI models can be time-consuming and resource-intensive
- Controlling output requires domain-specific knowledge
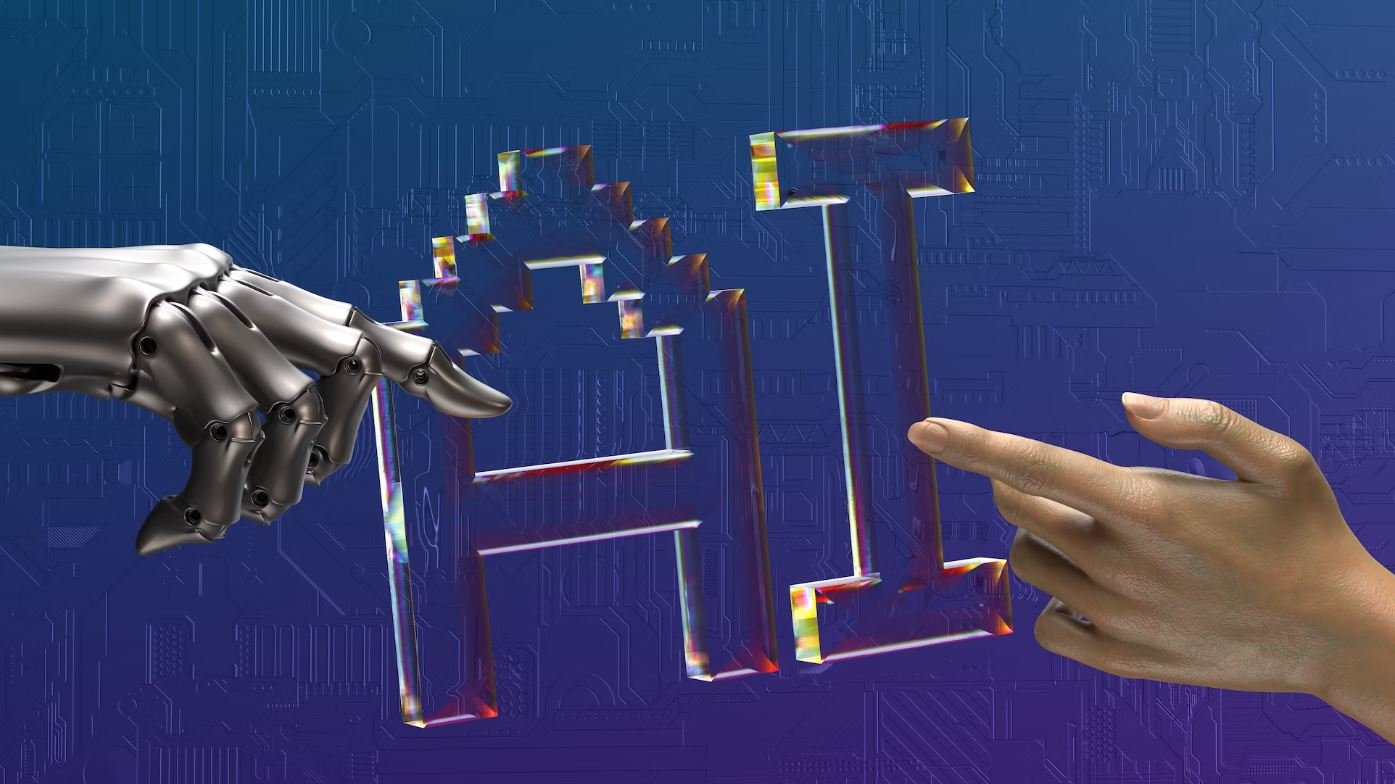
Table: Comparison of Generative AI Models
In this table, we compare the different generative AI models based on their capabilities, training requirements, and output quality. Each model offers unique strengths, but understanding these differences can help guide technological advancements and creative applications.
Generative AI Model | Capabilities | Training Requirements | Output Quality |
---|---|---|---|
Recurrent Neural Networks (RNN) | Text generation, music composition | Long training time, large datasets | Moderate quality, prone to repetition |
Generative Adversarial Networks (GAN) | Image synthesis, video generation | Long training time, labeled datasets | High quality, realistic output |
Variational Autoencoders (VAE) | Image reconstruction, anomaly detection | Moderate training time, unlabeled datasets | Variable quality, high diversity |
Table: Generative AI Applications by Industry
The potential applications of generative AI span across various industries. This table showcases how different sectors are utilizing generative AI to enhance creativity, improve productivity, and revolutionize traditional processes.
Industry | Generative AI Application |
---|---|
Entertainment | Virtual characters, scriptwriting assistance |
Fashion | Design inspiration, customized garments |
Healthcare | Medical imaging analysis, drug discovery |
Finance | Trading strategies, fraud detection |
Table: Generative AI Algorithms Performance Metrics
Assessing the performance of generative AI algorithms is crucial for evaluating their effectiveness and guiding further improvements. This table presents different metrics used to measure the performance of generative AI models.
Metric | Description | Optimal Value |
---|---|---|
Perplexity | Measure of language model confidence | Lowest possible value |
FID (Fréchet Inception Distance) | Comparison of generated and real image feature statistics | Close to zero |
Diversity | Extent of variation in generated outputs | High as possible, without compromising quality |
Table: Generative AI Success Stories
Generative AI has already led to remarkable achievements across various domains. In this table, we highlight some notable success stories that demonstrate the potential of this technology and its impact on society.
Domain | Success Story |
---|---|
Art | Sale of AI-generated artwork for $432,500 |
Music | AI-composed symphony performed by a live orchestra |
Healthcare | AI-generated drug molecule with high efficacy |
Table: Ethical Considerations in Generative AI Development
As generative AI evolves, ethical considerations become increasingly important. This table outlines key ethical concerns surrounding generative AI and emphasizes the need for responsible development and deployment of the technology.
Ethical Concern | Explanation |
---|---|
Bias and Discrimination | Inherent biases in training data reflected in generated content |
Intellectual Property | Ownership and copyright implications of AI-generated works |
Misinformation and Manipulation | Potential for AI-generated content to mislead or deceive users |
Table: Key Challenges in Generative AI
Developing generative AI systems involves tackling several challenges. This table highlights the major obstacles that researchers and developers face when attempting to push the boundaries of generative AI technology.
Challenge | Description |
---|---|
Data Quality and Quantity | Accessing and labeling massive datasets for training |
Performance Evaluation Metrics | Defining reliable metrics to assess the output quality |
Interpretability | Understanding and explaining the decision-making process |
Table: Public Attitudes Towards Generative AI
Understanding public sentiment towards generative AI is crucial for its acceptance and responsible implementation. This table presents data from surveys conducted to gauge public perception, concerns, and expectations regarding generative AI technology.
Survey Question | Responses (%) |
---|---|
Do you believe generative AI can be a force for positive change? | 82% Yes, 18% No |
Are you concerned about generative AI technology being misused? | 64% Yes, 36% No |
Would you trust a generative AI-generated recommendation? | 79% Yes, 21% No |
Table: Future Directions in Generative AI Research
Ongoing research in generative AI is shaping the direction of this field. This table presents emerging trends and promising avenues for further exploration, paving the way for exciting advancements in the future.
Research Direction | Description |
---|---|
Multi-modal Generation | Generating content across multiple domains simultaneously |
Controllable Generation | Enabling users to manipulate specific attributes of the output |
Improved Training Efficiency | Reducing the time and resources required for training |
Generative AI has shown incredible potential in various fields, enabling machines to create original content and push the boundaries of human imagination. This article explored different aspects of generative AI, from comparing models and applications to discussing ethical considerations and future research directions. With continuous innovation and responsible development, generative AI will continue to transform industries, enhance artistic expression, and shape the way we interact with intelligent machines.
Frequently Asked Questions
What is generative AI?
Generative AI refers to a branch of artificial intelligence (AI) that aims to create models or systems capable of generating new, creative, and realistic data, such as images, music, or text.
How does generative AI work?
Generative AI uses techniques such as deep learning and neural networks to train models on large datasets. These models learn the patterns, styles, and characteristics of the input data and can then generate new data that resembles the original examples.
What are the applications of generative AI?
Generative AI has a wide range of applications, including image and video synthesis, text generation, music composition, game development, virtual reality, and even drug discovery. It can be used in industries such as entertainment, marketing, healthcare, and design.
What are the benefits of generative AI?
Generative AI enables the creation of new and original content without human intervention, reducing the time and effort required for creative tasks. It can also assist in exploring new design spaces, generating personalized recommendations, and providing insights into complex systems.
Are there any risks associated with generative AI?
While generative AI offers immense potential, there are also risks to consider. Generated content can be used to spread misinformation, create deepfakes, or infringe on intellectual property rights. It is crucial to develop ethical guidelines, regulations, and responsible usage practices to mitigate these risks.
What are some popular generative AI models?
There are various popular generative AI models, including Generative Adversarial Networks (GANs), Variational Autoencoders (VAEs), and Transformers. GANs, for example, involve a competitive process between a generator and a discriminator to create realistic outputs.
How can generative AI be evaluated?
Evaluation of generative AI models can be challenging as there is no objective metric to measure creativity or quality. Common evaluation methods involve human judgment, user feedback, or comparison to existing datasets. Diversity, novelty, coherence, and fidelity are among the criteria used to assess the outputs.
What are the limitations of generative AI?
Generative AI models have some limitations, such as the potential to generate biased or offensive content based on the biases present in the training data. It can also struggle with long-term dependencies, producing outputs that lack context or coherence. Additionally, training generative AI models can be computationally expensive.
What is the future of generative AI?
As technology advances, we can expect generative AI to play a more significant role in various domains. It may enable personalized experiences, facilitate creative collaborations between humans and AI, assist in learning and education, and contribute to the development of AI-generated art and entertainment.
How can I get started with generative AI?
To get started with generative AI, you can learn about deep learning, neural networks, and generative models. Familiarize yourself with popular frameworks such as TensorFlow, PyTorch, or Keras. There are online courses, tutorials, and open-source projects available that can help you dive into the field and experiment with generative AI techniques.