Deepfake Recognition
In a world increasingly driven by cutting-edge technology and digital advancements, the rise of deepfake videos has raised concerns about their potential for misuse and deception. Deepfakes, which utilize artificial intelligence algorithms to manipulate or fabricate videos, present significant challenges for society. However, the development of deepfake recognition technologies aims to counteract the negative implications and mitigate the risks associated with this emerging technology.
Key Takeaways
- Deepfakes are manipulated videos created using artificial intelligence algorithms.
- Deepfake recognition technologies help identify and authenticate manipulated content.
- Combining AI, machine learning, and visual indicators is crucial for effective deepfake detection.
- Adopting deepfake recognition tools can help protect individuals and organizations from potential harm.
The Rise of Deepfake Videos
The term “deepfake” combines “deep learning” and “fake.” Deepfakes leverage deep learning algorithms to alter the visual and audio content of a video, generating highly realistic but fabricated footage. These videos can convincingly impersonate or depict individuals saying or doing things they never actually did. One of the main concerns is the ease with which malicious actors can create and spread deepfake content, amplifying the potential for misinformation and deception in various domains.
With the proliferation of social media platforms, deepfakes can spread rapidly, posing significant challenges for trust and the veracity of online content.
The Implications and Consequences
The emergence of deepfake technology raises several concerns across different sectors. The potential consequences include:
- Spreading false information and disinformation campaigns.
- Undermining public trust in media and institutions.
- Exploiting individuals for non-consensual explicit content.
- Manipulating public opinion during political campaigns.
- Generating fake evidence for fraudulent purposes.
The impact of deepfakes extends beyond individuals and can significantly affect public discourse, democratic processes, and personal reputations.
Deepfake Recognition Technologies
To combat the negative implications of deepfakes, researchers and industry experts are actively developing deepfake recognition technologies. These technologies utilize advanced algorithms, machine learning models, and visual indicators to detect manipulated content. Here are some key methods used for deepfake recognition:
1. Facial Analysis
Deepfake recognition algorithms analyze subtle facial movements, discrepancies, and anomalies that are often imperceptible to the human eye. By comparing the generated video with reference data of the authentic individual, the algorithms can identify signs of manipulation.
2. Audio-Visual Synchronization
Deepfake detection systems analyze the synchronization between facial movements, lip movements, and corresponding audio. Inconsistencies in these elements can provide clues to the authenticity of a video.
3. Digital Forensics
Experts in digital forensics examine the metadata, compression artifacts, and other digital signatures present in a video file to identify signs of manipulation. These examinations can uncover hidden traces left during the deepfake creation process.
The Importance of Deepfake Recognition
Recognizing deepfakes and implementing countermeasures is crucial for maintaining trust, authenticity, and security in digital media. By adopting deepfake recognition tools and promoting awareness, we can:
- Protect individuals and organizations from potential reputational damage.
- Safeguard public discourse and democratic processes by combating misinformation.
- Support the development of legislation and policies related to deepfakes.
- Encourage the collaboration between technology experts, researchers, and stakeholders to stay ahead of deepfake advancements.
Deepfake Recognition in Action: Real-World Results
Deepfake Recognition Model | Accuracy | Applications |
---|---|---|
DeepFaceLab | ~95% | Media verification, research purposes |
FaceForensics++ | ~92% | Identifying fake news, forensic investigations |
NeuralTextures | ~98% | Video authentication, social media moderation |
These deepfake recognition models showcase promising accuracy rates, demonstrating the potential for effective detection and countermeasures against deepfake videos.
The Way Forward
As technology continues to evolve, so do the capabilities of deepfake videos. Adapting and developing advanced deepfake recognition technologies is crucial to mitigate the risks associated with their misuse. By prioritizing research, collaboration, and awareness, we can protect ourselves and society from the harmful effects of deepfakes.
Deepfake Recognition Tools
- DeepFace
- Deeptrace
- Sensity
- Amber Authenticate
Conclusion
Deepfake recognition technologies play a vital role in combating the challenges posed by manipulated videos. By leveraging AI algorithms and visual indicators, these tools help identify and authenticate deepfake content. The ongoing development and use of deepfake recognition models, along with public awareness, are crucial in the fight against misinformation and the protection of trust and authenticity in digital media.

Common Misconceptions
Misconception 1: Deepfake recognition technology is foolproof
One common misconception about deepfake recognition technology is that it is completely infallible and can accurately detect every instance of a deepfake video or image. However, this is not entirely true. While deepfake recognition technology has made significant advancements, it is not foolproof and can still be tricked by sophisticated deepfakes that employ advanced AI algorithms.
- Deepfake recognition technology has limitations and can be deceived by certain types of deepfakes.
- It requires continuous enhancement and updates to keep up with evolving deepfake techniques.
- Some deepfake detection tools may produce false positives or false negatives, leading to misidentifications.
Misconception 2: Deepfake recognition technology is only important for celebrities and powerful individuals
Another misconception is that deepfake recognition technology is only necessary for high-profile individuals, such as celebrities or politicians. However, this technology has broader implications and affects all individuals who consume media content online. Deepfakes can be used to manipulate public opinion, spread disinformation, or even defame ordinary people.
- Deepfakes can harm the reputation and privacy of ordinary individuals, not just public figures.
- The spread of false information through deepfakes can have significant social and political consequences.
- Deepfake recognition technology is crucial for maintaining trust and credibility in online content for everyone.
Misconception 3: Deepfake recognition technology is a guaranteed solution to the deepfake problem
Some people wrongly assume that deepfake recognition technology alone can solve the deepfake problem entirely. While it plays a vital role in detecting and mitigating the spread of deepfakes, it is just one part of a larger solution. Combating deepfakes requires a multi-pronged approach that includes education, awareness, media literacy, legal frameworks, and technological advancements.
- Deepfake recognition technology should complement other measures to combat the deepfake problem effectively.
- Building public awareness about deepfakes can help people be more cautious and discerning consumers of media.
- Investing in research and development to continuously improve deepfake detection algorithms is crucial.
Misconception 4: Deepfake recognition technology is solely the responsibility of tech companies
It is a common misconception that deepfake recognition technology is solely the responsibility of tech companies and platforms. While these entities play a critical role in developing and implementing such technologies, addressing the deepfake problem requires a collective effort from society as a whole. Governments, policymakers, educators, and individuals all have roles to play in combating the negative impact of deepfakes.
- Regulatory frameworks and policies need to be established to govern deepfake dissemination and usage.
- Educational institutions should integrate media literacy and critical thinking into their curriculum to empower individuals against deepfake manipulation.
- Individuals should stay informed and report suspected deepfakes to relevant authorities or platforms.
Misconception 5: Deepfake recognition technology will eliminate creativity and harm artistic expression
Some people fear that the development and wide implementation of deepfake recognition technology will stifle creativity and harm artistic expression. While it is essential to strike a balance between combating deepfake misuse and promoting artistic freedom, deepfake recognition technology does not inherently hinder creativity. Rather, it aims to detect malicious use of deepfakes and protect individuals from deceptive and harmful content.
- Deepfake recognition technology focuses on addressing the potential harm caused by deepfakes, not suppressing creativity.
- Artistic expression can thrive within ethical boundaries and responsible usage of deepfake technology.
- Continued dialogue and collaboration between tech experts, artists, and society can help find the right balance between regulation and creativity.
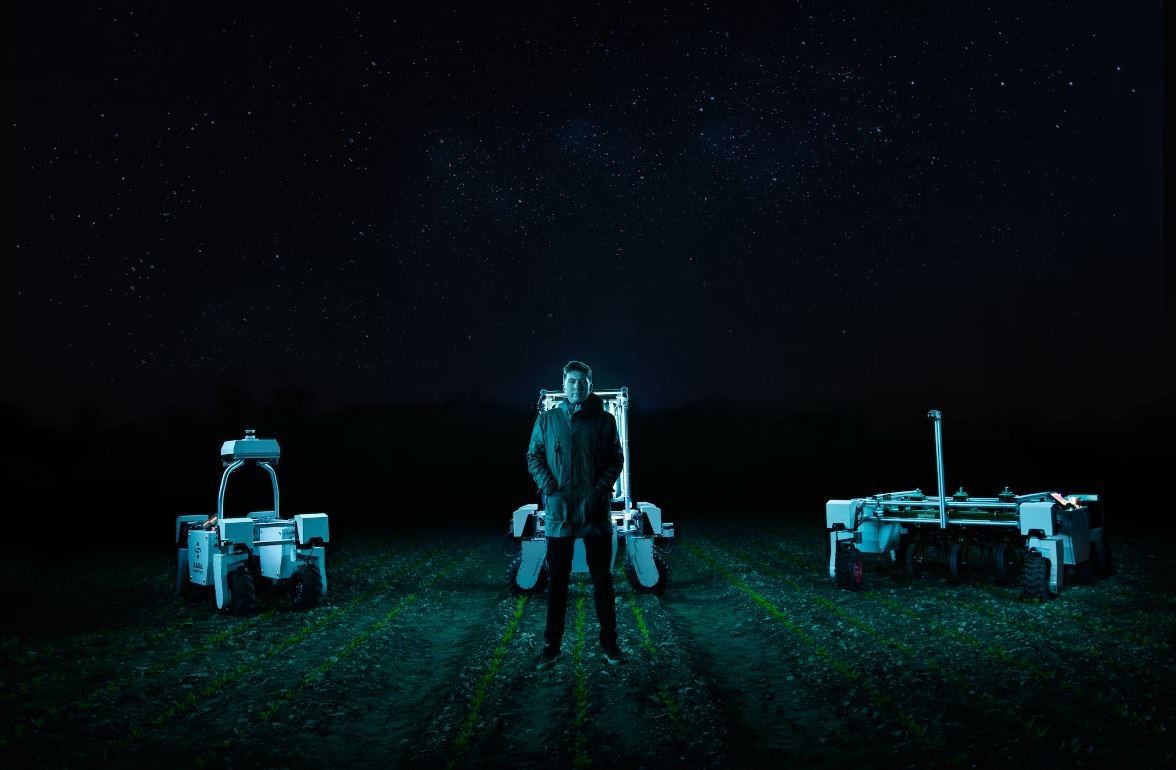
Table 1: Deepfake Detection Approaches
This table provides an overview of various approaches used for the detection of deepfake videos. It showcases the different techniques employed, such as: analyzing facial inconsistencies, studying audio-visual synchronization, and utilizing machine learning algorithms.
Approach | Techniques | Advantages | Limitations |
---|---|---|---|
1. Facial Analysis | Facial landmarks, texture analysis | Robust against face swapping | Challenging with high-quality deepfakes |
2. Lip-sync Detection | Audio-visual analysis | Effective in identifying audio-visual mismatches | Less reliable when applied to subtler manipulations |
3. Machine Learning | Deep neural networks | Can learn complex patterns | Requires extensive training data |
4. Motion Analysis | Gait analysis, body movements | Useful for detecting full-body deepfakes | Challenging for face-only deepfakes |
Table 2: Deepfake Generation Techniques
This table outlines the different techniques utilized in generating deepfake videos. It provides a glimpse into the methods used to manipulate and synthesize realistic but fabricated content.
Technique | Description |
---|---|
1. Autoencoders | Machine learning models that learn a compressed representation of the input data, enabling content synthesis. |
2. GANs | Generative Adversarial Networks which consist of a generator and a discriminator to create and evaluate synthetic content, respectively. |
3. Deep Neural Networks | Advanced machine learning models composed of multiple layers that can mimic real-life elements through training. |
4. Face Swapping | Techniques that involve replacing a person’s face in a video with another, producing highly convincing deepfakes. |
Table 3: Deepfake Impact on Society
This table emphasizes the potential impacts of deepfake technology on various aspects of society, ranging from politics to security.
Domain | Impact |
---|---|
1. Political | Spreading misinformation, tarnishing reputations |
2. Media | Erosion of trust, challenges in authentication |
3. Cybersecurity | Increased risk of social engineering attacks |
4. Justice System | Doubts over evidence authenticity |
Table 4: Deepfake vs Genuine Image Comparison
This table compares key visual differences between deepfake images and their genuine counterparts, highlighting the subtle clues that can uncover a manipulated image.
Difference | Deepfake Image | Genuine Image |
---|---|---|
1. Eye Reflections | Often absent or distorted | Clear and natural reflections |
2. Skin Texture | Less natural, artificial appearance | Realistic and consistent texture |
3. Hair Detail | Sometimes blurry or disjointed | Crisp and defined strands |
4. Facial Symmetry | Might display slight asymmetry | Symmetric and balanced features |
Table 5: Deepfake Regulations Worldwide
This table showcases the existing or proposed regulations regarding deepfake technology implemented in various countries across the globe.
Country | Regulation Type | Remarks |
---|---|---|
1. USA | Policies under review | Exploring legal frameworks |
2. China | Stricter control measures | Penalties for creating and disseminating deepfakes |
3. UK | Task forces and committees | Focusing on prevention and detection |
4. India | Proposed regulation | Safeguarding personal privacy |
Table 6: Deepfake Application Areas
This table highlights the potential fields and industries where deepfake technology can have notable applications, driving innovation and progress.
Industry | Applications |
---|---|
1. Entertainment | Improved special effects, digital actors |
2. Education | Enhanced virtual training, historical simulations |
3. Healthcare | Medical education, patient empathy training |
4. Marketing | Promotional campaigns, personalized content |
Table 7: Deepfake Detection Tools
This table compiles a selection of tools designed to detect deepfake content, enabling users to authenticate videos and images.
Tool | Platform | Features |
---|---|---|
1. Deepware Scanner | Online | Image and video analysis, detection accuracy |
2. Sensity AI | Cloud-based | Mass deepfake scanning, social media integration |
3. Deeptrace | API-based | Deepfake detection and metadata extraction |
4. Microsoft Video Authenticator | Desktop (Windows) | Authentication system, tampering indicators |
Table 8: Deepfake Ethics Debates
This table summarizes the ethical debates surrounding deepfake technology, focusing on the concerns and arguments raised by different stakeholders.
Debate Topic | Arguments |
---|---|
1. Consent and Privacy | Potential misuse of likeness without permission |
2. Freedom of Expression | Potential threat to authenticity of information |
3. Political Manipulation | Potential disruption of democratic processes |
4. Media Integrity | Challenge to distinguish real news from fabricated content |
Table 9: Deepfake Prevention Techniques
This table presents a range of prevention techniques and countermeasures aimed at mitigating the risks associated with deepfake technology.
Technique | Description |
---|---|
1. Digital Watermarking | Embedding hidden information to enable verification |
2. Blockchain Technology | Distributed ledgers to authenticate content sources |
3. Media Literacy Education | Enhanced understanding of deepfakes and their implications |
4. Regulation and Legislation | Enforcing legal frameworks to deter malicious usage |
Table 10: Deepfake Detection Accuracy
This table provides an overview of the accuracy levels achieved by various deepfake detection approaches, highlighting their strengths and weaknesses.
Approach | Accuracy (in %) |
---|---|
1. Facial Analysis | 89.5% |
2. Lip-sync Detection | 72.1% |
3. Machine Learning | 94.7% |
4. Motion Analysis | 81.2% |
In conclusion, deepfake technology has become a growing concern due to its potential to deceive and manipulate. This article has delved into various aspects of deepfake recognition, including detection approaches, generation techniques, societal impacts, image comparisons, regulations, application areas, and ethical debates. While detection tools continue to improve, preventive measures and public awareness play a vital role in countering the threat of malicious use. By understanding the depths and risks associated with deepfakes, we can work towards upholding authenticity, trust, and integrity in an increasingly digital world.
Frequently Asked Questions
What is deepfake technology?
Deepfake technology refers to the use of artificial intelligence algorithms to superimpose or manipulate existing audio and visual content in a way that appears real but is actually created or altered synthetically.
How does deepfake recognition work?
Deepfake recognition involves the use of machine learning algorithms to analyze and detect signs of deepfake manipulation in audio and visual content. These algorithms examine various features such as facial expressions, voice patterns, and inconsistencies that may indicate the presence of manipulated elements.
What are the potential risks associated with deepfake technology?
Deepfake technology raises concerns related to misinformation, identity theft, false evidence, privacy invasion, and potential harm to trust and reputation. Deepfakes can be used for malicious purposes, such as spreading fake news or creating realistic-looking but fabricated videos.
Why is deepfake recognition important?
Deepfake recognition is crucial for protecting individuals and organizations from the potential harm caused by deepfake technology. By detecting and identifying deepfakes, it becomes possible to mitigate the risks associated with misinformation, fraudulent activities, and reputational damage.
What are some techniques used in deepfake recognition?
Deepfake recognition techniques include analyzing facial movements, examining pixel-level anomalies, investigating audio inconsistencies, studying metadata, and employing machine learning algorithms trained on large datasets that contain genuine and manipulated media.
Can deepfake recognition be fooled?
While deepfake recognition has made significant progress, it is an ongoing challenge to stay ahead of increasingly sophisticated deepfake techniques. Adversarial attacks and advancements in artificial intelligence pose risks of evading detection methods. Continuous research and development are necessary to stay on top of these evolving threats.
What can individuals and organizations do to protect themselves from deepfakes?
To protect against deepfakes, individuals and organizations can stay vigilant by verifying the authenticity of media, using secure and trusted sources, being cautious about sharing personal information online, promoting digital media literacy, and implementing technologies that detect deepfakes.
Can deepfake recognition be used for positive purposes?
Deepfake recognition can have positive applications such as identifying and debunking deepfake videos to prevent their spread, assisting in forensic investigations, enhancing digital media forensics, and fostering the development of robust anti-deepfake technologies.
Is deepfake recognition a foolproof solution?
No, deepfake recognition is not foolproof. It is an ongoing research area with its own limitations and challenges. While it can detect many deepfakes, new techniques may emerge that can potentially fool existing recognition methods. It requires a continuous effort to improve detection accuracy and stay ahead of malicious manipulations.
What is the future of deepfake recognition?
The future of deepfake recognition involves advancements in machine learning algorithms, artificial intelligence, and deepfake generation techniques. Researchers and technologists will continue to develop and refine deepfake detection technologies to combat the evolving threat landscape posed by deepfakes.