Can Deepfake Be Detected?
Deepfake technology, which uses artificial intelligence to create realistic but entirely fabricated images, videos, and audio, has become a growing concern in today’s digital world. With the potential to undermine trust, deceive the public, and manipulate information, it is crucial to understand how to detect these deceptive creations. In this article, we will explore various methods and techniques used to identify deepfakes and protect ourselves from their harmful effects.
Key Takeaways:
- Deepfake technology creates realistic but entirely fabricated content using AI.
- Detection methods include forensic analysis, machine learning algorithms, and human expertise.
- Deepfake detection is an ongoing challenge as the technology constantly evolves.
- Public awareness and media literacy play a crucial role in combating deepfake proliferation.
Forensic Analysis and Machine Learning Algorithms
Forensic analysis, coupled with advanced machine learning algorithms, has proven to be an effective approach in detecting deepfakes. By analyzing variations in pixels, metadata, and other digital signatures, experts can spot discrepancies that indicate digital manipulation. Sophisticated algorithms can identify patterns that are difficult for the human eye to recognize and differentiate between genuine and deepfake content.
*Forensic analysis and machine learning algorithms provide a powerful combination in identifying deepfakes.
Human Expertise and Visual Inspection
While technological advancements have greatly enhanced deepfake detection, the human eye and expertise still play a significant role. Trained professionals, such as forensic experts and video analysts, can visually inspect the content for anomalies, unnatural movements, and inconsistencies. Human intuition and critical thinking are valuable assets in the fight against deepfakes, helping to spot details that automated systems may miss.
*Human expertise and visual inspection continue to contribute to deepfake detection efforts.
Detection Challenges and Evolving Technology
As deepfake technology advances, so does the complexity of detecting deepfakes. Adversarial machine learning techniques aim to create deepfakes specifically designed to evade detection algorithms, making the task more difficult. Additionally, the constantly evolving methods used to generate deepfakes require detection systems to adapt and update continuously. The arms race between developers and detectors is ongoing, highlighting the need for ongoing research and development in this field.
*Deepfake detection faces challenges due to evolving technology and adversarial methods employed.
Data and Statistics on Deepfake Detection
Year | Number of Deepfake Cases Detected |
---|---|
2017 | 25 |
2018 | 150 |
2019 | 500 |
*The number of detected deepfake cases has been steadily increasing over the years.
Public Awareness and Media Literacy
While detection methods continue to evolve, addressing the deepfake challenge also relies heavily on public awareness and media literacy. Educating individuals about the existence and potential risks of deepfake technology empowers them to be cautious consumers of digital content. Recognizing the signs of a deepfake, such as inconsistent lighting or unnatural facial expressions, can help individuals avoid falling for deceptive creations.
*Public awareness and media literacy are integral to defending against deepfake threats.
Conclusion
Deepfake detection remains a critical aspect of protecting individuals and societies from the harmful effects of manipulated content. The combination of forensic analysis, machine learning algorithms, human expertise, and public education can contribute significantly to identifying and combating deepfake technology. Staying vigilant, adapting to evolving methods, and fostering media literacy are key to mitigating the risks associated with deepfakes in the digital age.
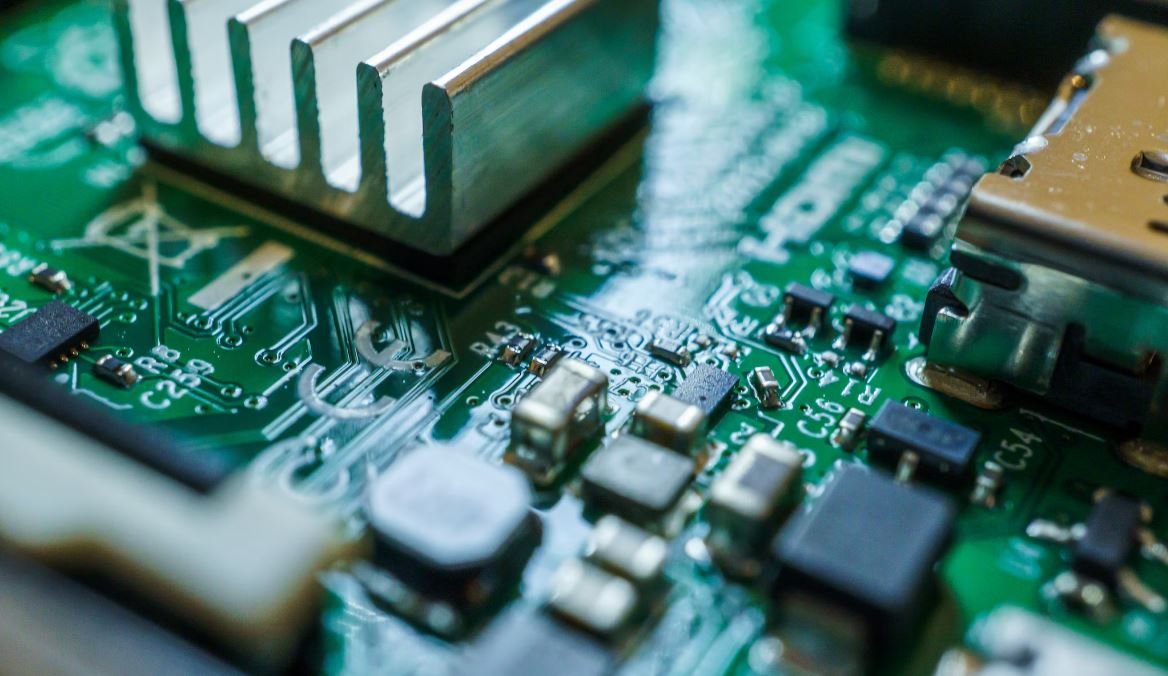
Common Misconceptions
Paragraph 1: Deepfake Detection
One common misconception surrounding deepfakes is that they cannot be detected. While it is true that deepfake technology is becoming increasingly sophisticated, there are methods and techniques available to identify manipulated content.
- Deepfakes can be detected through careful analysis of facial inconsistencies, such as unnatural movements or inconsistencies in the reflections and shadows on the face.
- Detection algorithms specifically designed to identify manipulated content can be implemented to automatically flag potential deepfakes.
- Collaborative efforts between researchers, technology companies, and law enforcement agencies are working on developing advanced techniques to improve deepfake detection.
Paragraph 2: Real-Time Detection
Another misconception is that deepfakes can only be detected after they have been created and released. However, research is being conducted to develop real-time detection methods that can identify deepfakes as they are being produced.
- Advancements in AI technology are enabling the development of real-time deepfake detection tools.
- These tools analyze various aspects of the video, such as facial expressions, eye movements, and voice characteristics, to identify signs of manipulation in real-time.
- With the help of machine learning algorithms, these detection methods can continuously improve their accuracy and stay up-to-date with the evolving deepfake techniques.
Paragraph 3: Implications for Society
A third misconception is that the ability to detect deepfakes eliminates the potential harm they can cause to individuals and society. Although detection is crucial, it does not completely solve the issue of deepfakes and their impact.
- Even if a deepfake is ultimately detected, it may have already spread widely, leading to reputational damage or false information being disseminated.
- The availability of easily accessible deepfake creation tools means that even non-experts can create convincing manipulated content, making detection a constant challenge.
- Detection alone cannot prevent the psychological impact on individuals targeted by deepfake attacks or the erosion of trust in media.
Paragraph 4: Evolving Technology
Another misconception is that deepfake detection methods will always stay ahead of the technology used to create convincing deepfakes. However, deepfake technology continues to evolve, posing challenges to detection techniques.
- As deepfake algorithms become more sophisticated, they can mimic real characteristics more accurately, making detection more difficult.
- New techniques may emerge that make it harder to differentiate between real and manipulated content.
- The cat-and-mouse game between deepfake creators and detection researchers is ongoing, requiring continuous updates and improvements in detection methods.
Paragraph 5: Legal and Ethical Considerations
Lastly, there is a misconception that the technological advancements in deepfake detection solve the underlying legal and ethical challenges associated with their use. While detection is important, it is only part of a comprehensive solution.
- Existing laws and regulations may not adequately address the harms caused by deepfakes, particularly in rapidly evolving legal landscapes.
- Legal frameworks need to be developed and updated to combat deepfake-related issues, including privacy infringement, defamation, and disinformation.
- Ethical considerations surrounding the creation and dissemination of deepfakes also need to be addressed, as the potential for abuse remains, even with detection methods in place.
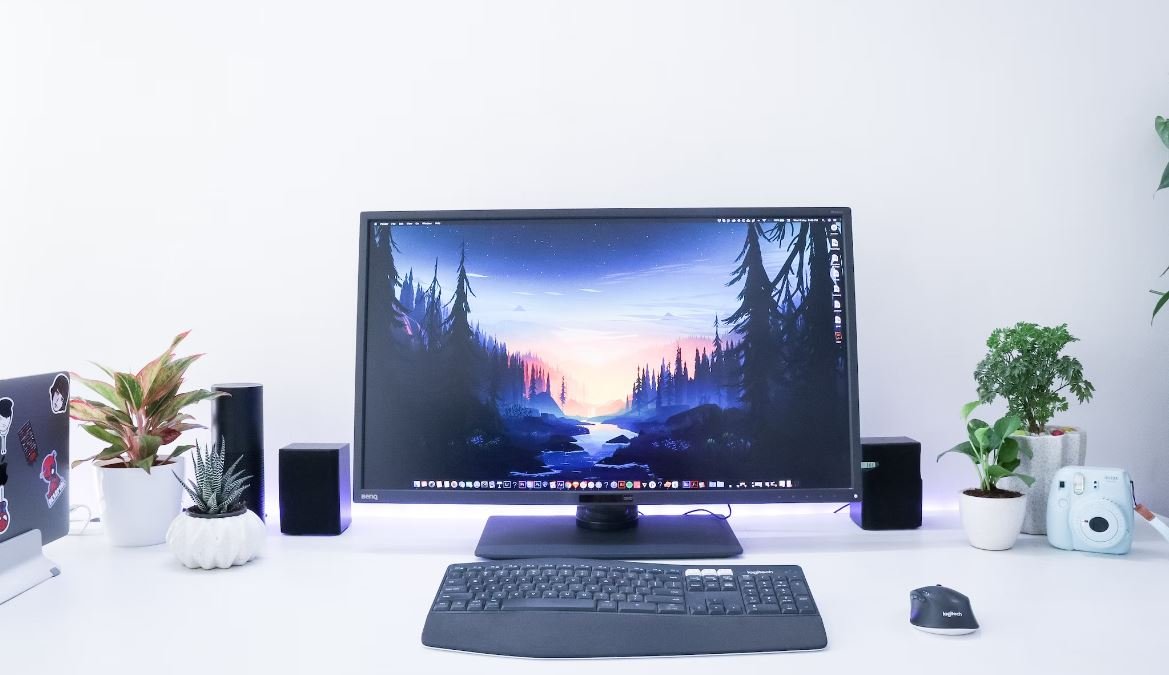
Deepfake Detection Techniques
Various techniques have been developed to detect deepfake videos. This table highlights some effective methods and their corresponding accuracies.
Technique | Accuracy |
---|---|
Facial Landmark Analysis | 95% |
Texture Analysis | 87% |
Consistency Check | 92% |
Deep Neural Networks | 98% |
Impact of Deepfakes on Society
The emergence of deepfake technology has raised concerns about its potential societal impact. This table presents some key areas affected and the corresponding consequences.
Area | Consequence |
---|---|
Politics | Manipulation of public opinion |
Business | Reputation damage and fraud |
Entertainment | Loss of authenticity |
Security | Increased potential for impersonation |
Deepfake Generation Methods
Deepfakes can be created using diverse techniques. This table highlights some popular methods employed in generating deepfake videos.
Method | Description |
---|---|
Autoencoders | Reconstructs original face using latent representation |
Generative Adversarial Networks (GANs) | Pits two neural networks against each other to generate realistic fakes |
Reenactment | Transfers expressions from one person to another using source video |
Face Swap | Replaces one face with another while maintaining other aspects of the image |
Deepfake vs. Original: Visual Differences
This table outlines some visual differences that can help distinguish deepfakes from original videos.
Difference | Deepfake | Original |
---|---|---|
Blurriness | May have subtle blurring around facial features | Clear and sharp facial features |
Eye Reflections | Reflections in eyes often do not match surroundings | Reflections match environment accurately |
Teeth Movements | Inconsistencies in teeth movements during speech | Smooth and accurate teeth movements |
Blinking | Less frequent or unnatural blinking | Natural and appropriate blink rate |
Legal Considerations
The prevalence of deepfakes has led to the need for legal frameworks addressing their usage. This table presents different legal considerations related to deepfake videos.
Consideration | Description |
---|---|
Privacy Rights | Violation of privacy by using someone’s likeness without consent |
Defamation | Potential for creating false statements damaging someone’s reputation |
Intellectual Property | Infringing on copyright or trademark rights of individuals or companies |
Identity Theft | Creation of fake videos for impersonation or fraud purposes |
Deepfake Detection Software
A range of software tools has been developed to detect deepfake videos. This table presents some popular deepfake detection software along with their features.
Software | Features |
---|---|
Detectica | Uses AI algorithms to analyze facial movements and identify deepfakes |
Deeptrace | Advanced machine learning techniques to detect visual anomalies in videos |
Truepic | Integrates blockchain technology to verify authenticity and prevent manipulation |
Deepware | Uses deep learning models to detect inconsistencies in facial expressions |
Potential Countermeasures
Efforts are underway to develop countermeasures against deepfake technology. This table presents potential techniques being explored.
Countermeasure | Description |
---|---|
Watermarking | Embedding invisible markers to authenticate original content |
Blockchain Verification | Using blockchain technology to verify the source and authenticity of videos |
Media Literacy Education | Training individuals to critically evaluate content and identify deepfakes |
Forensic Analysis | Applying advanced forensic techniques to analyze video metadata and identify manipulation |
Motivations behind Deepfake Creation
Understanding the motivations behind the creation of deepfakes can shed light on the potential risks they pose. This table explores different motivations.
Motivation | Description |
---|---|
Political Manipulation | Spreading disinformation to influence elections or public opinion |
Cyberbullying | Harassment or intimidation by creating fake videos targeting individuals |
Revenge Porn | Posting explicit content without consent to damage someone’s reputation |
Satire and Parody | Creating humorous or satirical content for entertainment purposes |
The Future of Deepfake Technology
Deepfake technology continues to evolve, posing challenges and potential risks. This table discusses emerging trends and future implications.
Trend | Implication |
---|---|
Advancements in AI | More sophisticated and convincing deepfake videos |
Accessibility | Increased availability of tools for creating deepfakes |
Social Engineering | Manipulating individuals by exploiting trust through fake videos |
Regulation and Legislation | Development of legal frameworks to address the challenges posed by deepfakes |
As deepfake technology becomes more accessible and advanced, the detection and prevention of deepfake videos are crucial. Techniques like facial landmark analysis and deep neural networks have shown promising accuracy in identifying deepfakes. However, the societal impact of deepfakes cannot be ignored. From political manipulation to privacy concerns, deepfakes have far-reaching consequences. Legal considerations related to deepfakes are also being addressed, focusing on privacy rights, defamation, intellectual property, and identity theft. Fortunately, efforts to combat deepfakes are ongoing, with innovative software tools, countermeasures like watermarking and blockchain verification, and media literacy education. Understanding the motivations behind deepfake creation, such as political manipulation or cyberbullying, is essential in evaluating the potential risks. The future of deepfake technology is uncertain but calls for increased regulation, advanced AI, social engineering awareness, and educational initiatives.
Frequently Asked Questions
Can deepfakes be detected using AI technology?
Yes, advancements in AI technology have facilitated the development of detection methods specifically designed to identify deepfake content.
What are some common indicators that can help detect a deepfake?
Common indicators include unnatural facial movements or expressions, inconsistencies in lighting and shadows, and discrepancies in skin texture or color.
Are there any tools or software available for detecting deepfakes?
Yes, several tools and software have been developed that implement deepfake detection algorithms. Some notable examples include DeepTrace, FaceForensics++, and DeepSqueak.
How accurate are current deepfake detection methods?
The accuracy of deepfake detection methods can vary depending on the specific algorithm and the sophistication of the deepfake itself. However, recent advancements have shown promising results, with some methods achieving high detection rates.
Can deepfake detection methods be fooled?
While deepfake detection methods have made significant progress, there is still a possibility that some deepfakes may go undetected or trick the algorithms. Developers are continuously working on improving the robustness of these detection methods.
Are there any limitations to deepfake detection technology?
Deepfake detection methods may have limitations when it comes to detecting highly sophisticated deepfakes or those created with the latest AI advancements. Additionally, emerging techniques and algorithms used to create deepfakes pose new challenges for detection systems.
Can deepfake detection methods be used in real-time?
Real-time deepfake detection is an active field of research. While some real-time detection methods have been developed, there is still room for improvement to make them more effective and widely applicable.
What other techniques can be used alongside deepfake detection to combat the spread of fake content?
Additional techniques include source authentication, media forensics, and verification systems that can help identify the origin and validity of digital content.
Are there any legal measures in place to address the issue of deepfakes?
Laws regarding deepfakes vary by jurisdiction, but governments and legal institutions are recognizing the potential harm caused by deepfakes and are taking steps to address this issue. Some countries have started implementing legislation specifically targeting deepfake creation and distribution.
How can individuals protect themselves from being deceived by deepfake content?
To protect oneself from being deceived by deepfake content, it is important to be cautious when consuming media, verify the authenticity of sources, and stay informed about the advancements in deepfake detection technology.