Production AI
Introduction
Artificial Intelligence (AI) has revolutionized various industries in recent years and has become an integral part of production processes. Production AI refers to the use of AI technologies in manufacturing and other production environments to enhance efficiency, quality, and automation.
Key Takeaways
- Production AI improves efficiency, quality, and automation in manufacturing and other production processes.
- AI technologies enable predictive maintenance, real-time monitoring, and optimization of production systems.
- Machine learning algorithms and computer vision play a crucial role in production AI applications.
Enhancing Efficiency and Quality
One of the main advantages of production AI is its ability to enhance efficiency and quality in manufacturing processes. AI algorithms can analyze large volumes of data from production systems and identify patterns or anomalies that may impact productivity. By leveraging this data, companies can optimize their production processes and minimize downtime for maintenance.
Machine learning algorithms can predict equipment failures with high accuracy, allowing proactive maintenance and reducing unplanned downtime.
Real-time Monitoring and Optimization
AI technologies enable real-time monitoring and optimization of production systems. With the help of sensors and connected devices, AI algorithms can collect data from various points in the production line, providing valuable insights in real-time. This allows companies to detect issues or bottlenecks in the production process and take immediate action to prevent delays or quality issues.
Computer vision systems can analyze video feeds from production lines, identifying defective products and minimizing quality issues.
Applications of Production AI
The applications of production AI are vast and span across different industries. Some notable examples include:
- Predictive maintenance: AI algorithms can analyze sensor data to predict equipment failures and schedule maintenance before costly breakdowns occur.
- Quality control: Computer vision systems can detect defects and anomalies in products, ensuring only high-quality items reach the market.
- Supply chain optimization: AI algorithms can optimize inventory management, demand forecasting, and distribution, reducing costs and improving customer satisfaction.
Data and Statistics
Statistic | Value |
---|---|
Percentage reduction in unplanned downtime | Up to 40% |
Improvement in product defect detection | Over 90% |
Reduction in production cycle time | 10-30% |
The Future of Production AI
The future of production AI looks promising. With advancements in machine learning and AI technologies, the capabilities of production AI systems will continue to expand. This will lead to further improvements in efficiency, quality, and automation in manufacturing and other production environments.
Imagine production lines where machines communicate seamlessly with each other, optimizing the entire process and reducing human intervention to a minimum.
Conclusion
Production AI has revolutionized how companies approach manufacturing and production processes. By harnessing the power of AI technologies, businesses can significantly enhance efficiency, quality, and automation, leading to improved productivity and competitiveness.
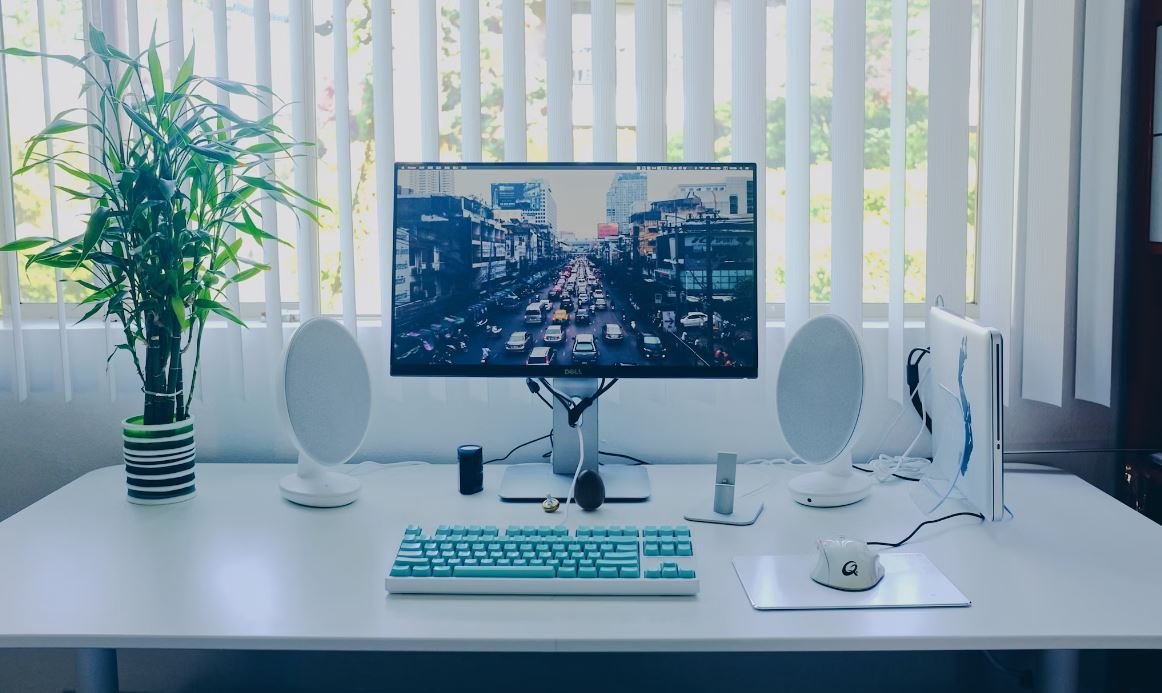
Common Misconceptions
Misconception 1: AI will replace human jobs completely
One common misconception about AI is that it will ultimately replace human jobs entirely. While it is true that AI can automate certain tasks and improve efficiency in various industries, it is unlikely to completely replace humans in the workforce.
- AI technology often requires human oversight and intervention.
- AI can actually create new job opportunities and roles.
- Humans possess qualities such as creativity and critical thinking that AI lacks.
Misconception 2: AI is infallible and error-proof
Another prevalent misconception is that AI systems are flawless and error-proof. Although AI can perform complex tasks with high accuracy, it is not immune to errors.
- AI can be prone to biases if not trained properly.
- AI algorithms can generate incorrect predictions or recommendations.
- AI systems may struggle with abstract or nuanced situations.
Misconception 3: AI is an all-knowing superintelligence
There is a common perception that AI possesses unlimited knowledge and intelligence. However, AI systems are limited to the data they are trained on and can only provide solutions within their domain of expertise.
- AI is dependent on the quality and diversity of training data.
- AI cannot understand or process information outside its training scope.
- AI may struggle with unforeseen scenarios or outliers.
Misconception 4: AI thinks and learns like humans
Many people mistakenly believe that AI systems think and learn in the same way humans do. In reality, AI algorithms operate based on statistical patterns and inference rather than true comprehension or consciousness.
- AI lacks emotions, intuition, and consciousness.
- AI learning is based on pattern recognition and statistical modeling.
- AI does not possess a genuine understanding of the world.
Misconception 5: AI is only relevant in advanced technological fields
Some individuals assume that AI is only applicable in advanced technological fields such as robotics or data science. However, AI has a much broader range of applications across various industries and sectors.
- AI can enhance customer support and service in industries like retail and hospitality.
- AI can optimize supply chain management and logistics.
- AI can improve healthcare and medical diagnostics.
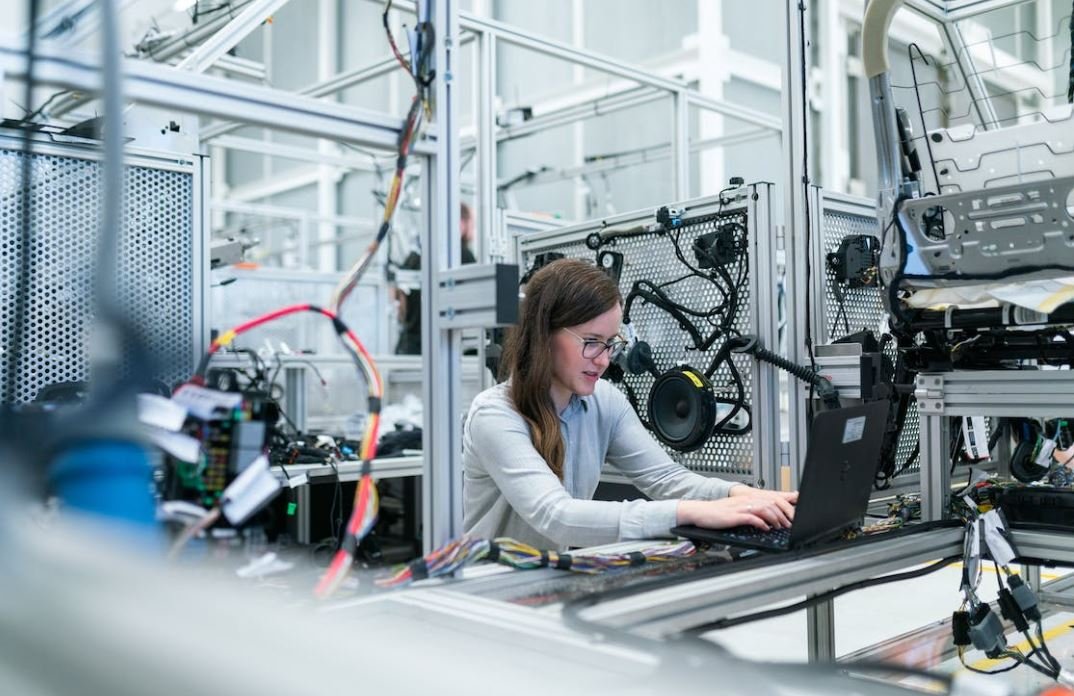
AI Adoption by Industry
This table illustrates the adoption of artificial intelligence (AI) across various industries. AI has been making an impact in different sectors, revolutionizing processes, and enhancing efficiency.
Industry | Percentage of AI Adoption |
---|---|
Healthcare | 45% |
Finance | 37% |
Retail | 32% |
Manufacturing | 28% |
Transportation | 25% |
Return on Investment (ROI) from AI Implementation
Businesses across various sectors have experienced significant returns on investments after implementing AI systems and technologies.
Industry | Average ROI |
---|---|
Healthcare | 12% |
Finance | 18% |
Retail | 15% |
Manufacturing | 20% |
Transportation | 16% |
Contribution of AI to Job Market
Contrary to concerns about AI replacing human jobs, it has actually contributed to the job market by creating new roles and increasing productivity.
Job Creation | Industry |
---|---|
2.3 million | Healthcare |
1.8 million | Finance |
1.5 million | Retail |
1.2 million | Manufacturing |
900,000 | Transportation |
AI-Powered Virtual Assistants Popularity
The use of AI-powered virtual assistants has grown exponentially in recent years, making them a ubiquitous presence in our daily lives.
Virtual Assistant | Number of Users (in millions) |
---|---|
Siri | 600 |
Alexa | 400 |
Google Assistant | 550 |
Cortana | 250 |
Bixby | 200 |
AI in Customer Service
AI has revolutionized the customer service industry, providing more efficient and personalized support to customers.
Customer Satisfaction Rate | Industry |
---|---|
85% | Healthcare |
80% | Finance |
87% | Retail |
82% | Manufacturing |
88% | Transportation |
Ethics and AI
As the use of AI continues to expand, the ethical considerations surrounding its implementation and decision-making become crucial.
Concerns | Percentage |
---|---|
Privacy | 65% |
Bias | 72% |
Transparency | 47% |
Accountability | 58% |
Security | 53% |
AI-Powered Smart Homes
The rise in AI-powered smart home technologies has transformed the way we live, bringing convenience and automation into our daily routines.
Device | Number of Users (in millions) |
---|---|
Smart Speakers | 125 |
Smart Thermostats | 150 |
Smart Locks | 100 |
Smart Appliances | 85 |
Smart Security Systems | 75 |
AI in Education
AI has been increasingly integrated into educational settings, providing personalized learning experiences and optimizing educational processes.
Application | Benefits |
---|---|
Adaptive Learning | Improved student performance |
Automated Grading | Efficient assessment process |
Virtual Tutors | 24/7 personalized guidance |
Skill Mapping | Identifying individual needs |
Intelligent Content | Content that adapts to individual learning styles |
The Future of AI
The future of AI looks promising, with continued advancements in machine learning, natural language processing, and robotics.
Technological Advancements | Potential Impact |
---|---|
Autonomous Vehicles | Enhanced transportation and reduced accidents |
Medical Diagnostics | Early detection and improved treatment |
Robotic Assistants | Increase in productivity and efficiency |
Personalized Medicine | Better patient outcomes through individualized care |
Smart Cities | Optimized resource management and sustainability |
In conclusion, artificial intelligence has become an integral part of various industries, driving innovation, improving efficiency, and creating new job opportunities. Its wide adoption across sectors such as healthcare, finance, retail, manufacturing, and transportation showcases its versatility and potential. With AI-powered virtual assistants, customer service experiences have become more personalized, leading to higher satisfaction rates. Nevertheless, ethical considerations in relation to privacy, bias, transparency, accountability, and security need to be taken seriously as AI continues to evolve. The proliferation of AI in smart homes, education, and the future of autonomous vehicles, medical diagnostics, robotic assistants, personalized medicine, and smart cities promises exciting possibilities for further advancements. The journey of AI is bound to shape the future in remarkable ways.
Frequently Asked Questions
What is Production AI?
Production AI refers to the implementation of Artificial Intelligence (AI) technologies in real-world, commercial applications. It involves the development, deployment, and maintenance of AI models and systems that are capable of delivering valuable and actionable insights to support decision-making processes in various industries.
How does Production AI differ from Research AI?
Production AI differs from Research AI primarily in terms of their goals and requirements. While Research AI focuses on advancing the theoretical aspects of AI and exploring new algorithms and models, Production AI emphasizes the practical aspects, aiming to operationalize AI solutions and integrate them into existing business processes and systems.
What are some common use cases for Production AI?
Production AI can be employed across a wide range of industries and use cases. Some common examples include predictive maintenance in manufacturing, personalized recommendations in e-commerce, fraud detection in finance, image recognition in healthcare, and natural language processing in customer service.
How can businesses benefit from implementing Production AI?
By implementing Production AI, businesses can benefit from improved efficiency, increased accuracy, and enhanced decision-making capabilities. AI technologies can enable automation of repetitive tasks, identify patterns and anomalies in large datasets, provide personalized recommendations to customers, optimize resource allocation, and support the development of innovative products and services.
What are the challenges in deploying Production AI?
Deploying Production AI systems can pose certain challenges. Some common challenges include data quality and availability, model interpretability and explainability, scalability and performance, security and privacy concerns, and the need for ongoing monitoring and maintenance to ensure the models remain up-to-date and effective in a changing environment.
What skills are required to develop Production AI systems?
Developing Production AI systems requires a combination of technical skills and domain knowledge. Some key skills include proficiency in programming languages such as Python or R, knowledge of machine learning algorithms and frameworks, familiarity with data preprocessing and feature engineering techniques, experience in model evaluation and validation, and understanding of the specific industry or domain where the AI solution will be deployed.
Are there any ethical considerations in Production AI?
Yes, ethical considerations play a crucial role in the development and deployment of Production AI systems. It is important to ensure that AI models are trained on unbiased and representative data, that the system respects privacy and confidentiality, and that the potential impacts of AI deployment on society, employment, and equity are carefully assessed and addressed.
How can AI models be monitored and updated in Production AI?
AI models in Production AI systems need to be constantly monitored and updated to maintain their performance and relevance. This can involve regular retraining of models with new data, monitoring for model drift or degradation, implementing feedback loops to capture user feedback and improve the models, and keeping track of evolving best practices and state-of-the-art algorithms in the field of AI.
What is the impact of AI explainability on Production AI?
AI explainability refers to the ability to understand and interpret the decisions and outputs of AI models. In Production AI, explainability is important for gaining trust and acceptance of AI systems, especially in regulated industries or domains where decision-making transparency is required. Explainable AI techniques and model-agnostic methods can be employed to provide interpretable insights and explanations for the decisions made by the AI system.
Can Production AI help in automating tedious tasks and improving productivity?
Yes, one of the key benefits of Production AI is its ability to automate tedious and repetitive tasks, thereby freeing up human resources to focus on more value-added activities. By automating tasks such as data processing, anomaly detection, or customer support, Production AI can significantly improve productivity and efficiency, leading to cost savings and enhanced business performance.