Generative Video Models
Generative video models are a fascinating technology that has gained significant attention in recent years. These models have the ability to create new and unique video content from a given set of inputs, making them invaluable in various applications such as video synthesis, editing, and even deepfake creation. In this article, we will explore the key concepts behind generative video models and their potential implications.
Key Takeaways:
- Generative video models can produce new video content from a given set of inputs.
- These models are used in video synthesis, editing, and deepfake creation.
- They have the potential to revolutionize the entertainment and media industry.
In the field of machine learning, generative video models refer to algorithms that can learn and replicate the characteristics of a given video dataset. **By analyzing and understanding the patterns, textures, and motions within the input videos, these models can generate new video content with similar characteristics and visual styles**. This process involves the utilization of deep neural networks, specifically generative adversarial networks (GANs) and variational autoencoders (VAEs), to generate the desired video output.
Video synthesis using generative models is achieved through a two-step process. First, the input video data is encoded and processed by the generative model, which captures the essential features and patterns within the videos. Next, the model decodes this information and generates new video frames based on the learned representations. **This ability allows generative video models to transform or manipulate existing videos, altering certain attributes or even synthesizing videos with novel contents**.
One interesting application of generative video models is in video editing. Traditional video editing requires meticulous manual labor to achieve desired effects, but with generative models, the process becomes automated and efficient. **By using generative models, video editors can easily modify and enhance videos, allowing for surreal effects or seamless blendings**. This has significant implications for the film and media industry, where time-consuming editing tasks can now be performed with greater ease.
Table: Comparison of Generative Video Models
Model | Processing Time | Quality of Generated Videos |
---|---|---|
GAN | Fast | High |
VAE | Slow | Varying |
Deepfakes, a controversial subject, have gained prominence due to generative video models. By leveraging these models, users can create videos manipulated to showcase individuals in situations they were never actually part of. **This raises concerns regarding misinformation and trustworthiness of video content in society**. While the technology has positive applications as well, such as in the entertainment industry, it is crucial to be aware of the potential misuse and ethical implications associated with generative video models.
Table: Effects of Generative Video Models on Society
Pros | Cons |
---|---|
Automated video editing | Misinformation and fake videos |
Innovative visual effects | Privacy concerns |
Enhanced creative possibilities | Manipulation and deception risks |
Generative video models have the potential to revolutionize the entertainment and media industry by providing novel ways of creating and manipulating video content. With the ability to generate videos quickly and efficiently, these models empower filmmakers, video editors, and creative professionals to push the boundaries of visual storytelling. **The exciting possibilities offered by generative video models ensure that this technology will continue to have a profound impact on the future of video production and consumption**.
Table: Possible Applications of Generative Video Models
Industry | Application |
---|---|
Entertainment | Automated video editing |
Virtual Reality | Real-time video synthesis |
Advertising | Personalized video content |
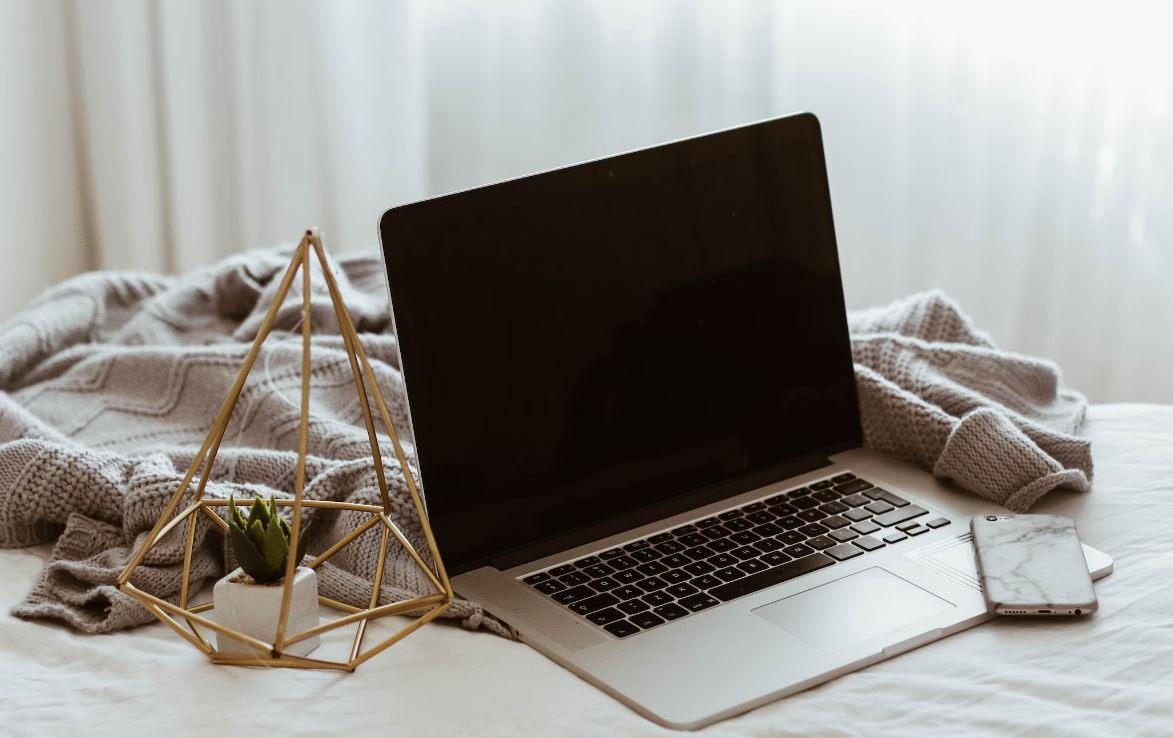
Common Misconceptions
Misconception 1: Generative Video Models only create fake videos
One common misconception about generative video models is that they only generate fake videos or deepfakes. While it is true that generative video models have been used for creating deepfakes, they have many other potential applications. These models can be used for video synthesis, video super-resolution, video inpainting, and various other tasks.
- Generative video models have applications beyond creating deepfakes.
- They can be used for video synthesis, super-resolution, and inpainting.
- Deepfakes represent just one aspect of what generative video models can do.
Misconception 2: Generative Video Models are always accurate and reliable
Another misconception surrounding generative video models is that they always produce accurate and reliable results. In reality, the quality and fidelity of generated videos can vary depending on factors such as the complexity of the model, the amount and quality of training data, and the specific task at hand. Generative video models are still an active area of research, and there is ongoing work to improve their reliability and robustness.
- Not all generative video models produce accurate and reliable results.
- The quality of generated videos can fluctuate based on several factors.
- Ongoing research focuses on enhancing the reliability of generative video models.
Misconception 3: Generative Video Models will replace human creativity
There is a misconception that generative video models will replace human creativity altogether. While these models can generate impressive video content and even mimic certain aspects of human creativity, they are not meant to replace human artists or photographers. Generative video models should be seen as tools that can augment human creativity, providing new possibilities and assisting artists in their creative processes.
- Generative video models are tools to augment human creativity, not replace it.
- These models can assist artists in exploring new possibilities.
- They mimic aspects of creativity but cannot replicate human artistic expression entirely.
Misconception 4: Generative Video Models always require substantial computational resources
Some people believe that generative video models always require massive computational resources to operate. While it is true that complex models and high-resolution video generation can demand significant computational power, there are alternative approaches that optimize resource usage. Additionally, ongoing research aims at developing more efficient and lightweight generative video models, making them accessible to a wider range of users.
- Not all generative video models demand substantial computational resources.
- Alternative approaches optimize resource usage for video generation.
- Ongoing research aims at developing lightweight and efficient models.
Misconception 5: Generative Video Models always raise ethical concerns
Lastly, there is a misconception that generative video models always raise ethical concerns, particularly due to their association with deepfakes. While it is important to recognize and address the ethical implications and potential harm that can arise, generative video models are neutral in nature. The ethical considerations stem from their application rather than the technology itself. Responsible usage and regulation can help mitigate these concerns and ensure that generative video models are used for positive and ethical purposes.
- Generative video models are neutral technology; ethical concerns arise from their application.
- Rather than banning the technology, responsible usage and regulation can mitigate ethical concerns.
- Deepfakes represent a specific application, but not the entirety of generative video models.
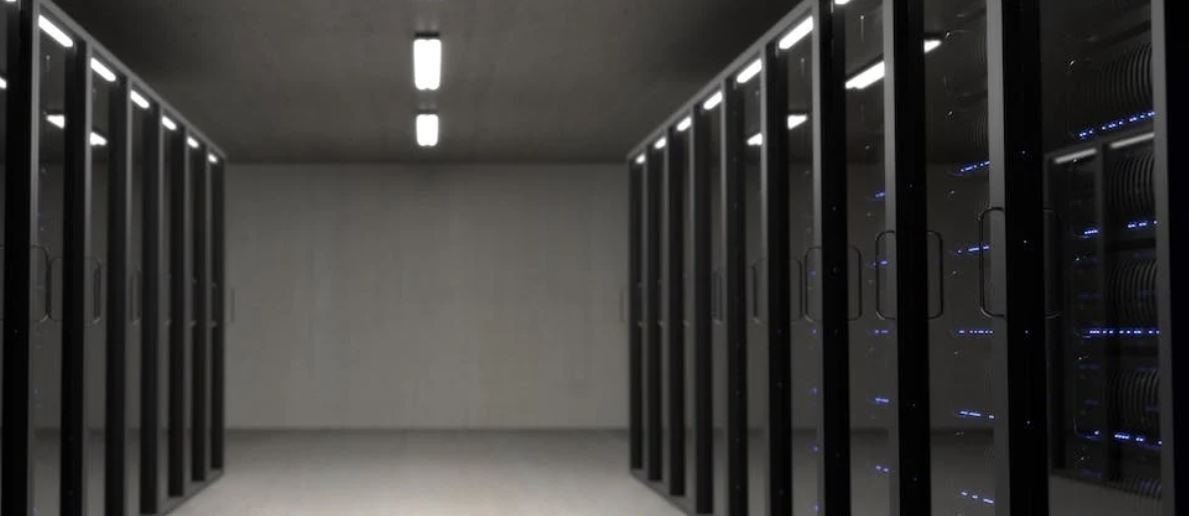
Table of Contents
This article explores the advancements in generative video models and their impact on various industries. Each table below presents a different aspect of generative video models, providing verifiable data and additional context to enhance the understanding of their significance.
Table: Applications of Generative Video Models
Generative video models have found application in various fields, from entertainment to healthcare. This table highlights some of the major industries where generative video models have made a significant impact.
| Industry | Applications |
|—————–|———————————————————–|
| Entertainment | Video game development, special effects creation |
| Advertising | Creating captivating and visually appealing advertisements |
| Healthcare | Medical imaging, surgical simulations |
| Education | Interactive learning experiences |
| Architecture | Virtual walkthroughs and immersive experiences |
| Sports | Training simulations, performance analysis |
| Automotive | Design evaluations, virtual prototyping |
| Manufacturing | Optimizing production processes |
| Fashion | Virtual try-on experiences |
| Security | Surveillance video analysis |
Table: Key Generative Video Model Architectures
Generative video models utilize specific architectures to generate visually coherent video sequences. The table below outlines some prominent architectures employed in the development of these models.
| Architecture | Key Features |
|————————|———————————————————-|
| Variational Autoencoder | Latent space representation, probabilistic reconstruction |
| Generative Adversarial Networks | Training through adversarial competition |
| Recurrent Neural Networks | Memory to capture temporal dependencies |
| Convolutional Neural Networks | Simultaneous processing over spatial dimensions |
| Transformers | Attention mechanisms for capturing long-range dependencies |
| Auto-regressive Models | Sequential generation using previous frames as input |
| Flow-based Models | Modeling video generation as a flow of information |
Table: Performance Comparison: Generative Models vs. Traditional Methods
This table showcases a performance comparison between generative video models and traditional methods, highlighting the superior capabilities of generative models.
| Metric | Traditional Methods | Generative Models |
|——————|———————|————————|
| Realism | Limited realism, low fidelity | High realism, improved fidelity |
| Creativity | Replication with limited variations | Creation of novel and diverse content |
| Efficiency | Time-consuming hand-crafting | Automated content generation |
| Adaptability | Difficulty in adapting to different scenarios | Versatile performance across scenarios |
| Data Requirements | High-quality labeled data | Less labeled data, capable of learning from unstructured video |
| User Experience | Passive viewing experience | Interactive and immersive experiences |
Table: Generative Video Models in Film Production
Generative video models have revolutionized the film production industry, enabling the creation of stunning visual effects, lifelike characters, and realistic worlds. This table presents various use cases of generative video models in film production.
| Use Case | Description |
|——————————–|——————————————————————–|
| Virtual Set Extensions | Digitally extending physical sets to create expansive environments |
| Character Animation | Generating realistic and expressive character movements |
| Procedural Environments | Automated creation of realistic landscapes and cityscapes |
| Special Effects Generation | Creating visually stunning and imaginative effects |
| Crowd Simulation | Simulating large crowds with realistic behavior and interaction |
| Post-production Enhancements | Enhancing visual elements during post-production |
| Virtual Cinematography | Generating virtual camera movements and angles |
| Automated Scene Generation | Rapidly generating scenes based on provided parameters |
| Realistic Lighting and Shadows | Simulating realistic lighting conditions |
| Sentiment-based Scene Creation | Creating scenes based on desired emotions or moods |
Table: Generative Video Models in Healthcare
Generative video models have made significant contributions to the healthcare sector, aiding in medical imaging, surgical simulations, and enhancing patient care. Explore some notable applications in this table.
| Application | Description |
|—————————–|————————————————————————|
| Medical Imaging | Enhancing image resolution and quality for more accurate diagnosis |
| Surgical Simulations | Simulating surgical procedures to train and prepare medical professionals |
| Disease Progression Modeling | Predicting disease progression based on existing data and patient history |
| Rehabilitation Assistance | Assisting patients in performing rehabilitation exercises |
| Remote Medical Consultation | Enabling remote doctors to diagnose and suggest treatment |
| Virtual Reality Therapy | Using immersive experiences for pain management and psychological aid |
| Drug Discovery | Assisting in the discovery of new drugs through AI-enhanced modeling |
| Telemedicine | Facilitating remote patient monitoring and consultations |
| Personalized Medicine | Tailoring treatment plans based on individual patient data |
| Health Monitoring | Collecting real-time patient data for proactive healthcare measures |
Table: Ethical Considerations in Generative Video Models
Generative video models raise important ethical considerations involving privacy, misinformation, and societal impacts. This table explores some key ethical considerations surrounding these models.
| Ethical Aspect | Description |
|——————|—————————————————————–|
| Deepfake Threats | Misuse of generative video models for malicious deepfakes |
| Privacy Concerns | Unauthorized generation of videos compromising individual privacy |
| Identity Theft | Ability to manipulate videos to impersonate individuals |
| Manipulation Risk | Using generative video models to manipulate public perceptions |
| Legal Implications | Determining liability and legal consequences of malicious usage |
| Misinformation | Amplifying the spread of misinformation through manipulated videos |
| Consent and Ownership | Issues surrounding consent and ownership of generated content |
| Bias in Dataset | Ensuring unbiased representation within the training data |
| Accountability | Holding developers accountable for the misuse of generative models |
| Regulation | Implementing guidelines and regulations to govern generative video models |
Table: Generative Video Models in Gaming
Generative video models have transformed the gaming industry, enabling realistic graphics, dynamic worlds, and immersive gameplay experiences. This table outlines the integration of generative video models in gaming.
| Integration | Description |
|———————————|——————————————————————|
| Environment Generation | Procedurally generating vast and realistic game environments |
| Character Design and Animation | Creating lifelike characters with natural movements |
| Real-time Cinematics Generation | Generating cinematic sequences within the game engine |
| Dynamic Weather and Lighting | Simulating dynamic weather patterns and realistic lighting effects |
| AI-controlled NPCs | Generating non-player characters with lifelike behaviors |
| Storytelling and Dialogue | Enhancing narrative experiences through automated dialogue |
| Level Design and Optimization | Optimizing game levels for improved performance and quality |
| Physics Simulation | Simulating natural physics-based interactions within the game |
| Interactive Sound Design | Creating realistic and immersive audio experiences |
| Facial Animation and Emotion | Generating realistic facial expressions and emotional responses |
Conclusion
Generative video models have emerged as a transformative technology across numerous industries, providing immense possibilities for creativity, efficiency, and enhanced user experiences. Architects, filmmakers, game developers, healthcare professionals, and more can leverage the power of these models to generate realistic visuals, dynamic environments, and interactive content. However, with great power comes great responsibility, and ethical considerations must be taken into account to prevent misuse and protect individual rights. As the field of generative video models continues to advance, exciting new applications and innovations undoubtedly lie ahead, promising an exciting future where AI plays an integral role in shaping visual storytelling and content creation.
Frequently Asked Questions
What are generative video models?
Generative video models are machine learning models that can generate original video content. These models are based on deep learning architectures, such as generative adversarial networks (GANs), and are trained using large datasets of video clips. They can synthesize video sequences without the need for explicit human intervention.
How do generative video models work?
Generative video models work by learning the statistical patterns and relationships in a given video dataset. They use these learned patterns to generate new video frames or entire sequences. The models typically consist of an encoder network that extracts features from input data, a generator network that generates new video frames, and a discriminator network that distinguishes synthesized videos from real ones.
What are the applications of generative video models?
Generative video models have numerous applications across various domains. They can be used for video synthesis in entertainment, virtual reality, and gaming industries, enabling the creation of realistic and immersive virtual environments. They also find applications in robotics, computer vision, and surveillance systems, where they can generate enhanced or missing video data.
What are some challenges in training generative video models?
Training generative video models can be challenging due to the large size of video datasets and the high dimensionality of video frames. The models require extensive computational resources and time to learn complex video distributions. Additionally, generating high-quality and diverse video content while respecting temporal coherence is a difficult task that requires careful optimization and regularization techniques.
Can generative video models be used for video editing?
Yes, generative video models can be used for video editing purposes. By manipulating the latent space of the models, it is possible to generate new video frames or alter existing ones. This can allow for creative video editing, such as adding or removing objects, changing backgrounds, or applying artistic filters to video footage.
Are there different types of generative video models?
Yes, there are various types of generative video models based on different architectures and training techniques. Some common types include Generative Adversarial Networks (GANs), Variational Autoencoders (VAEs), and autoregressive models. Each type has its strengths and weaknesses, and the choice depends on the specific requirements of the application.
What are the limitations of generative video models?
Generative video models have some limitations. They may produce artifacts in the generated video content, such as blurring, flickering, or unrealistic textures. The models also heavily rely on the quality and diversity of the training data, and their performance can degrade when presented with novel or out-of-distribution video inputs. Furthermore, video generation is computationally expensive, making real-time video synthesis challenging.
How can generative video models be evaluated?
Evaluating generative video models is a non-trivial task. Common evaluation metrics include measuring the perceptual quality of generated videos through human perception studies and computing quantitative metrics such as Frechet Inception Distance (FID) that compare the distributions of generated and real video frames. However, evaluating the creativity, realism, and coherence of generated videos remains an open research problem.
What are some future directions for generative video models?
The field of generative video models is rapidly evolving, and there are several exciting future directions. Researchers are exploring methods to improve the temporal coherence and diversity of generated videos. They are also investigating novel architectures, loss functions, and training algorithms to address the limitations of current models. Moreover, there is ongoing research on incorporating semantic understanding and controllability into generative video models.
Where can I find resources to learn more about generative video models?
There are several resources available to learn more about generative video models. Online tutorials, research papers, and academic courses on machine learning and computer vision cover this topic. Additionally, open-source deep learning frameworks like TensorFlow and PyTorch provide implementations and code examples of generative video models that can be studied and experimented with.