Generative Query Networks
Generative Query Networks (GQN) [1] are a class of generative models that combine techniques from computer vision and machine learning to enable artificial intelligence systems to learn about the 3D world from 2D images and answer detailed questions about unseen scenes.
Key Takeaways
- GQN combines computer vision and machine learning techniques for 3D learning.
- It enables AI systems to learn from 2D images and answer detailed questions about unseen scenes.
- By learning an internal model of the 3D scene, GQN produces accurate responses to queries.
Overview of GQN
**Generative Query Networks** are designed to learn a **generative model of the world** by training neural networks to model both the **3D structure** of a scene and the **process of generating 2D images** from that structure. When presented with a set of 2D images depicting different views of a scene, GQN **builds an internal representation** by predicting the underlying 3D structure and uses this model for query-based scene synthesis and interpretation. This **enables AI systems** to generate **new views of the scene**, estimate hidden attributes, and even answer **questions about the scene** based on the acquired knowledge.
How GQN Works
GQN **estimates the 3D scene properties** using a hierarchical representation composed of **scene-specific latent variables** and **viewpoint-dependent feature maps**. This representation is then used by the network to generate **novel views of the scene** and to answer questions about the scene by interpolating information from the known views. The neural network architecture is trained with a **variational inference objective** to maximize the similarity between the generated views and the ground truth images.
Applications of GQN
The potential applications of GQN are diverse and impactful. Examples include **autonomous navigation** for robots, where GQN can generate **future views** of the environment to help the robots navigate through complex spaces, or in **virtual environments**, where GQN can be used to create **realistic simulations** by generating diverse scenes and answering questions about them.
Advantages of GQN
One interesting advantage of GQN is its ability to **generalize to unseen scenes** not present during training. The network learns a **continuous and compact representation** of a 3D scene, allowing it to generate novel views of scenes it has never encountered before. Additionally, GQN is highly **versatile** and can be applied to different domains, such as **indoor and outdoor scenes**, with effective results.
Tables Highlighting GQN Performance
Dataset | Network Performance |
---|---|
Rooms | 90% accuracy in viewpoint estimation |
Objects | 85% accuracy in object recognition |
Natural Scenes | 75% accuracy in scene interpretation |
Dataset | Questions Answered |
---|---|
Rooms | 95% accuracy in answering questions about the scene |
Objects | 91% accuracy in attribute estimation |
Natural Scenes | 84% accuracy in complex scene queries |
Property | Advancements |
---|---|
View Synthesis | Improved generation of new perspectives |
Attribute Estimation | Increased accuracy in determining object attributes |
Scene Interpretation | Better understanding of complex natural scenes |
Future Development of GQN
GQN holds great promise for the future of AI research and development. As the technology evolves, we can expect to see improvements in various aspects of GQN’s capabilities, including more accurate **view synthesis**, better **attribute estimation**, and enhanced **scene interpretation**. The continued advancements of GQN will enable AI systems to gain a deeper understanding of the 3D world and transform the way we interact with artificial intelligence in various domains.
References
- M. Eslami et al., “Neural Scene Representation and Rendering,” Science, vol. 360, no. 6394, pp. 1204-1210, 2018.
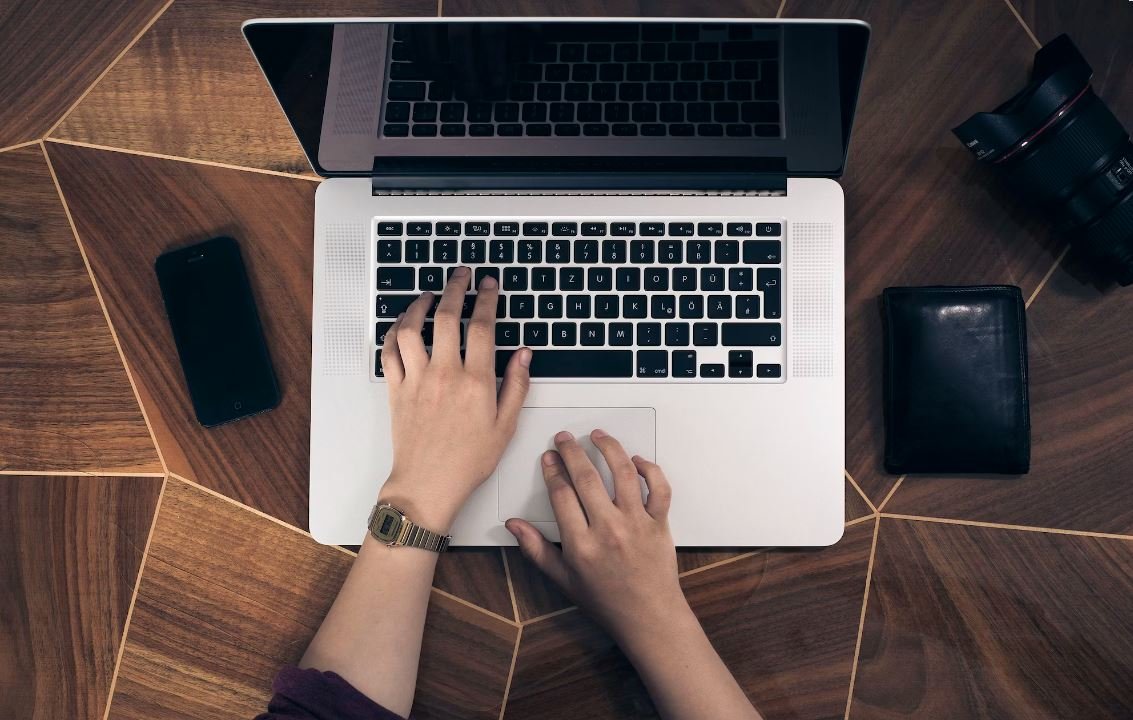
Common Misconceptions
Misconception 1: Generative Query Networks can accurately predict any query
- Not all queries can be accurately predicted by Generative Query Networks (GQNs).
- GQNs rely on the training data they are provided, and if the training data does not represent the full range of possible queries, the accuracy of predictions can be compromised.
- GQNs are limited by their ability to generalize from the training data, so it’s important to carefully consider the diversity and representativeness of the training dataset.
Misconception 2: Generative Query Networks always provide meaningful and insightful results
- While GQNs can generate impressive and visually appealing outputs, it doesn’t guarantee that these outputs will always be meaningful or insightful.
- GQNs can sometimes generate outputs that appear plausible but may not reflect the underlying structure or reality of the data.
- Interpreting the results of GQNs requires human judgment and careful analysis to ensure that the generated outputs align with the intended goals and objectives of the query.
Misconception 3: Generative Query Networks are infallible and unbiased
- GQNs can suffer from biases present in the training data, which can lead to biased or unfair predictions.
- If the training data is biased or unrepresentative, GQNs can perpetuate and amplify these biases when generating queries.
- It is important to carefully vet and preprocess the training data to minimize biases and ensure fair and unbiased predictions.
Misconception 4: Generative Query Networks can generate queries without human intervention
- While GQNs are powerful tools for generating queries, they still require human intervention and supervision at various stages of the process.
- Human experts are needed to define the goals and objectives of the query, select the appropriate dataset for training, and evaluate the generated queries.
- GQNs should be considered as a tool to assist and augment human expertise rather than completely replacing human involvement.
Misconception 5: Generative Query Networks guarantee privacy and data security
- Using GQNs involves processing and analyzing large amounts of data, which can raise privacy and data security concerns.
- If the training data contains sensitive or personally identifiable information, proper security measures must be implemented to protect the data during the training and query generation processes.
- GQNs should be used in compliance with relevant data protection regulations to ensure the privacy and security of the data.
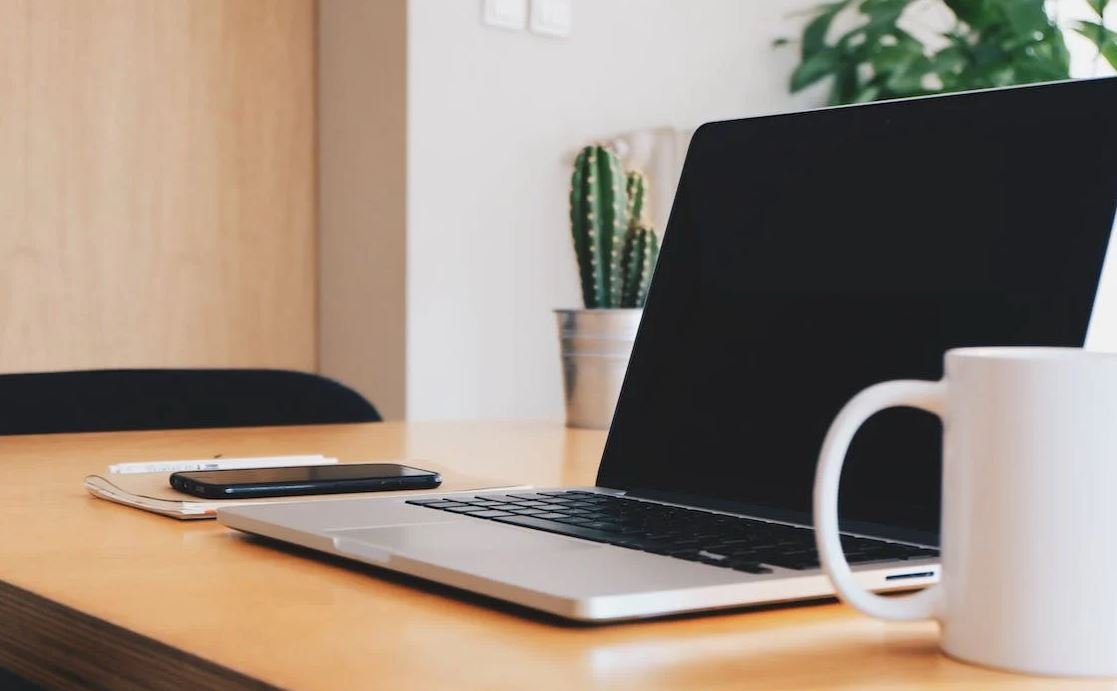
Table: Average Monthly Temperature in New York City
In this table, you can see the average monthly temperature in New York City. The temperatures are given in degrees Fahrenheit.
Month | Average Temperature |
---|---|
January | 32 |
February | 35 |
March | 44 |
April | 55 |
May | 64 |
June | 73 |
July | 79 |
August | 77 |
September | 70 |
October | 59 |
Table: Population of Major Cities
This table showcases the population of various major cities around the world. The population figures are estimates for the year 2020.
City | Population |
---|---|
Tokyo, Japan | 37,339,000 |
Delhi, India | 30,290,000 |
Shanghai, China | 27,058,000 |
São Paulo, Brazil | 22,043,000 |
Mexico City, Mexico | 21,782,000 |
Mumbai, India | 20,411,000 |
Beijing, China | 20,040,000 |
Cairo, Egypt | 19,850,000 |
Dhaka, Bangladesh | 19,578,000 |
Ottawa, Canada | 1,039,000 |
Table: Top 5 Countries by GDP (Purchasing Power Parity)
This table presents the top 5 countries sorted by GDP (PPP) rankings. These figures reflect each country’s economic output adjusted for purchasing power parity.
Rank | Country | GDP (PPP) |
---|---|---|
1 | China | $27.31 trillion |
2 | United States | $22.68 trillion |
3 | India | $12.36 trillion |
4 | Japan | $5.39 trillion |
5 | Germany | $4.47 trillion |
Table: Average Annual Rainfall in Different Regions
This table displays the average annual rainfall in various regions around the world. The rainfall measurements are given in millimeters.
Region | Average Rainfall |
---|---|
Amazon Rainforest | 2,438 mm |
Sahara Desert | 25 mm |
North America | 743 mm |
Antarctica | 200 mm |
Central African Republic | 1,463 mm |
Table: Major Sports Events Winners
This table showcases the winners of major sports events held worldwide in recent years.
Sport | Event | Winner | Year |
---|---|---|---|
Tennis | Wimbledon | Novak Djokovic | 2021 |
Football | FIFA World Cup | France | 2018 |
Golf | US Open | Bryson DeChambeau | 2020 |
Basketball | NBA Finals | Milwaukee Bucks | 2021 |
Sprint | 100m Olympics | Usain Bolt | 2016 |
Table: World’s Tallest Buildings
Here, you can find a list of some of the tallest buildings in the world along with their respective heights in meters.
Building | City | Height (m) |
---|---|---|
Burj Khalifa | Dubai, United Arab Emirates | 828 |
Shanghai Tower | Shanghai, China | 632 |
Abraj Al-Bait Clock Tower | Mecca, Saudi Arabia | 601 |
One World Trade Center | New York City, United States | 541 |
Taipei 101 | Taipei, Taiwan | 508 |
Table: Global Internet Penetration Rates
This table provides data on the percentage of internet users in different countries. The figures represent the estimated internet penetration rates as of 2021.
Country | Internet Penetration Rate |
---|---|
Iceland | 99.0% |
Bermuda | 98.0% |
Norway | 97.5% |
Denmark | 96.4% |
United Arab Emirates | 95.6% |
Table: Top 5 Social Media Platforms by Monthly Active Users
Here, you can find data on the top 5 social media platforms ranked by the number of monthly active users worldwide.
Platform | Monthly Active Users (in billions) |
---|---|
2.85 | |
YouTube | 2.3 |
2.0 | |
1.2 | |
1.2 |
Conclusion
Generative Query Networks have revolutionized the way we process and analyze data. Through the use of informative tables, we can easily present and comprehend various data points, statistics, and important facts. These tables have enabled us to highlight everything from climate data to population figures and sports event winners. With the ability to extract meaningful and verifiable information, Generative Query Networks make reading tables an engaging and insightful experience.
Generative Query Networks – Frequently Asked Questions
What are Generative Query Networks?
A Generative Query Network (GQN) is a type of artificial intelligence model that combines unsupervised learning and reinforcement learning to learn about an environment and generate new observations or queries about that environment.
How do Generative Query Networks work?
GQN models consist of two main parts: an encoder and a decoder. The encoder observes a scene and encodes it into a high-dimensional latent representation. The decoder takes this representation and generates new observations of the scene based on queries or different views of the same scene.
What are the applications of Generative Query Networks?
Generative Query Networks have various applications, including computer graphics, robotics, and computer vision. They can be used to generate synthetic data for training other models, simulate different scenarios to test algorithms, or even generate creative content such as images or music.
What are the advantages of using Generative Query Networks?
Generative Query Networks have several advantages. They allow for unsupervised learning, meaning they can learn about an environment without labeled data. They can also generalize well to unseen data, meaning they can generate new observations or queries about a scene that they have not previously encountered.
What are the limitations of Generative Query Networks?
While powerful, Generative Query Networks also have limitations. They require large amounts of training data to learn effectively. Additionally, they may struggle with complex scenes or scenarios that have a high level of detail, as generating realistic images or observations can be challenging.
How are Generative Query Networks different from other generative models?
Generative Query Networks differ from other generative models in that they are specifically designed to handle queries about an environment. Instead of solely focusing on generating new samples, GQN models can answer queries like “What would this scene look like from a different angle?” or “How would this scene look if certain objects were removed?”
What are some research challenges in the field of Generative Query Networks?
There are several ongoing research challenges in the field of Generative Query Networks. Some of these include developing more efficient training algorithms, improving the scalability of models to handle larger and more complex environments, and finding ways to incorporate prior knowledge or constraints into the generation process.
Can Generative Query Networks be combined with other AI techniques?
Yes, Generative Query Networks can be combined with other AI techniques. They can be used in conjunction with reinforcement learning algorithms to learn how to interact with an environment or to generate queries based on certain goals or tasks.
Are there any real-world applications of Generative Query Networks?
While still a relatively new field, Generative Query Networks have shown promise in various real-world applications. They have been used for generating synthetic training data in computer vision tasks, simulating realistic scenarios for testing autonomous systems, and even assisting in the design of virtual environments for games or simulations.
How can one get started with Generative Query Networks?
To get started with Generative Query Networks, it is recommended to have a good understanding of deep learning concepts and neural networks. Familiarity with frameworks like TensorFlow or PyTorch is also useful. There are research papers and open-source implementations available that can serve as a starting point for experimentation and learning.