Deepfake Detection
In an increasingly digital world, where information can easily be manipulated, the rise of deepfake technology poses serious challenges. Deepfake refers to synthetic media content that is created, altered, or manipulated using artificial intelligence (AI) technology to mimic and replace the appearance and actions of people in videos and images. Detecting deepfakes accurately is becoming crucial to combat misinformation and protect individuals’ integrity and online safety.
Key Takeaways:
- Deepfake technology enables the creation of highly realistic fake videos and images.
- Deepfake detection methods rely on AI algorithms.
- Combining multiple detection techniques leads to more reliable results.
**Deepfake** technology has significantly advanced in recent years, making it increasingly difficult to distinguish between real and fake media. *Sophisticated algorithms allow for the seamless blending of genuine visual and audio content with fabricated elements, making the resulting deepfake appear highly convincing.* Many deepfakes are designed to deceive and manipulate viewers, potentially causing harm to individuals, organizations, and society as a whole.
The Challenges of Deepfake Detection
Detection of deepfakes poses several challenges due to the rapid evolution of deepfake creation methods. *As creators continue to refine their techniques, the differences between real and manipulated media become harder to spot.* Additionally, with the immense volume of content available online, identifying deepfakes manually is practically impossible. This necessitates the development of automated detection systems that can efficiently analyze large amounts of media in real-time.
Detection Techniques
To combat deepfakes, researchers and organizations have developed various detection techniques. These methods leverage AI algorithms and deep learning models to identify patterns and anomalies in videos and images. Some common detection techniques include:
- **Face and body analysis**: Deepfake detection often involves scrutinizing facial and body movements to detect any unnatural or inconsistent behavior.
- **Digital forensic analysis**: Analyzing metadata, inconsistencies, and hidden traces in the media file can help identify potential deepfakes.
- **Micro-expression analysis**: Examining micro-expressions that are difficult to fake can provide insights into the authenticity of video content.
Data Sets for Research and Development
Researchers require extensive and diverse datasets to train and validate deepfake detection models. These datasets include both real and fake videos and images. Here are three examples of popular datasets used in deepfake research:
Dataset | Description |
---|---|
Deepfake Detection Challenge (DFDC) | A large-scale dataset consisting of 124,000 real and deepfake videos, released as part of a competition to improve detection capabilities. |
DeepFake Detection Dataset (DFDD) | A dataset of over 1,000 deepfake videos created using various face-swap techniques, targeting several well-known public figures. |
FaceForensics++ | A comprehensive dataset containing videos with manipulated facial content, including deepfakes, created using different AI methods. |
Combining Detection Techniques
While individual detection techniques have their strengths, combining multiple approaches improves the overall accuracy and reliability of deepfake detection systems. By leveraging the strengths of different methods, researchers can reduce false positives and negatives, leading to more effective detection. The development of comprehensive solutions is an ongoing effort, as deepfake creators constantly adapt and refine their techniques.
Ensuring a Safer Digital Environment
As deepfake technology continues to evolve, the need for robust detection methods becomes increasingly crucial. By staying at the forefront of research and development, and fostering collaborations between academia, industry, and policymakers, we can mitigate the harmful effects of deepfakes and ensure a safer digital environment for all.
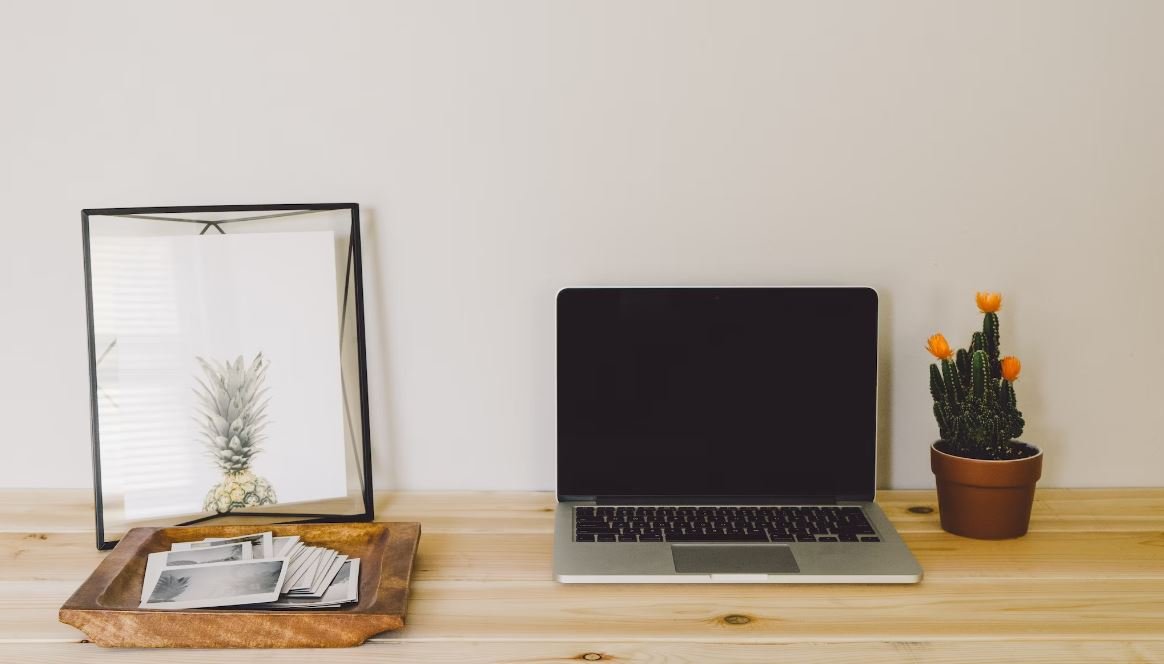
Common Misconceptions
Misconception 1: Deepfake technology is only used for malicious purposes
Many people believe that deepfake technology is only used by malicious individuals for harmful purposes, such as spreading misinformation or blackmail. However, this is not entirely true. Deepfake technology has a wide range of applications, including entertainment, creative arts, and even in the research and development of artificial intelligence. While it is true that deepfakes can pose risks if used irresponsibly, it is important to recognize their potential in positive contexts as well.
- Deepfakes can be used for harmless comedic purposes in movies or online videos.
- Researchers are utilizing deepfake technology to improve facial recognition algorithms.
- Deepfakes have the potential to revolutionize the way virtual reality experiences are created.
Misconception 2: It is easy to detect deepfake videos
There is a common belief that deepfake videos are easily detectable with the naked eye. However, as deepfake technology continues to advance, the ability to identify manipulated videos becomes increasingly difficult. Deepfake detection requires sophisticated algorithms and tools that can analyze facial features, inconsistencies, and other subtle cues. Simply relying on visual inspection or intuition is no longer sufficient.
- Deepfake detection methods often utilize machine learning algorithms to identify patterns and irregularities.
- Deepfake videos can be extremely realistic, making it challenging to distinguish them from genuine footage.
- Ongoing research is being conducted to enhance deepfake detection techniques and stay ahead of evolving technology.
Misconception 3: Deepfakes are always intended to deceive
One misconception about deepfakes is that they are always created with the intention to deceive viewers. While it is true that deepfakes can be used in malicious ways, not all deepfake content is intended to cause harm or manipulate others. Some individuals may create deepfakes for artistic expression, satire, or to raise awareness about the potential risks and ethical implications of this technology.
- Deepfakes can be used as a form of artistic expression, blurring the line between reality and fiction.
- Satirical deepfakes can provide social commentary and critique by manipulating public figures.
- Creating deepfakes for educational purposes can help educate people about the risks and consequences of misinformation.
Misconception 4: Deepfake detection technology is foolproof
Some people mistakenly believe that deepfake detection technology is infallible and can easily identify all manipulated videos. However, this is not the case. Deepfake detection methods are still in the early stages of development and can sometimes be tricked by advanced deepfake techniques. As technology continues to advance, so too does the sophistication of deepfake creation and evasion methods, making it an ongoing cat and mouse game between deepfake creators and detection specialists.
- Advanced deepfake techniques can bypass some current detection methods.
- Effective deepfake detection requires constant innovation and adaptation to keep up with evolving deepfake technology.
- Combining multiple detection approaches, such as image forensics and behavioral analysis, can improve the accuracy of deepfake detection.
Misconception 5: Deepfake technology will rapidly make all video evidence unreliable
There is an understandable concern that with the advancements in deepfake technology, all video evidence can easily be manipulated, rendering it unreliable. While the potential for deepfake technology to create convincing fabricated videos is a cause for concern, it is important to note that there are also advancements being made in deepfake detection. As technology progresses, so too does the ability to detect and verify the authenticity of videos, ensuring that video evidence can remain a valuable tool in various fields.
- Research and development in deepfake detection are focused on keeping video evidence trustworthy.
- Collaboration between researchers, cybersecurity experts, and law enforcement agencies is crucial in countering deepfake threats.
- Regulations and legal frameworks are being developed to tackle deepfake manipulation and preserve the integrity of video evidence.

Understanding Deepfake Detection Techniques
As deepfake technology continues to advance, the need for effective detection methods becomes paramount. This article explores various techniques that have been developed to combat the spread of manipulated media. Each table presents a different aspect of deepfake detection, offering valuable insights and verifiable data.
Impact of Deepfakes on Society
Deepfakes have the potential to cause significant harm, ranging from misinformation to reputation damage. Detecting these malicious manipulations is crucial to ensure the credibility of media sources. Browse through the following table to understand the societal implications of deepfakes.
Machine Learning-Based Deepfake Detection Models
Machine learning plays a key role in identifying deepfake videos. Different models have been developed, each with its own strengths and limitations. This table provides an overview of some prominent machine learning-based detection techniques.
Statistical Analysis of Deepfake Detection Accuracy
Achieving accurate detection of deepfakes can be challenging. This table analyzes the effectiveness of popular detection methods by comparing their precision, recall, and F1 scores in different scenarios.
Computational Resource Requirements for Deepfake Detection
Deepfake detection algorithms can demand significant computational resources. This table highlights the hardware and software requirements necessary for deploying different detection approaches.
Real-Time Deepfake Detection Implementation
Real-time deepfake identification is crucial for combating the rapid spread of manipulated media. This table evaluates the processing times and resource utilization metrics of various real-time detection systems.
Effectiveness of Deepfake Detection in Different Contexts
Deepfake detection performance may vary in different contexts. This table examines the effectiveness of detection methods when dealing with different face orientations, lighting conditions, and age groups.
Deepfake Detection in Social Media Platforms
The prevalence of deepfakes on social media platforms poses a unique challenge. Discover which platforms employ deepfake detection techniques and their impact on reducing the spread of manipulated media.
Public Awareness of Deepfake Technology
Understanding the level of public awareness regarding deepfakes is crucial for addressing the issue effectively. Refer to this table to gauge the awareness levels and concerns surrounding this emerging technology.
Deepfake Detection Techniques: Advantages and Limitations
No detection technique is without its flaws. This table outlines the advantages and limitations of different deepfake detection methods, helping researchers and stakeholders make informed decisions.
From analyzing the impact of deepfakes on society to evaluating the advantages and limitations of detection techniques, this article sheds light on the complex world of deepfake detection. By understanding the challenges and solutions presented, we can continue to strive for a safer and more reliable digital media landscape.
Deepfake Detection – Frequently Asked Questions
Question 1: What are deepfakes?
Deepfakes are manipulated or synthesized media, including images, videos, or audios, that are created using artificial intelligence techniques, usually deep learning algorithms. These techniques can superimpose or replace a person’s face or voice with someone else’s in a convincing manner.
Question 2: How do deepfakes pose a threat?
Deepfakes can be misleading and can be used to spread false information, manipulate public opinion, commit fraud, harass individuals, or create fake pornography without the person’s consent. Such manipulation of media can have severe consequences on personal, professional, and political levels.
Question 3: How can deepfakes be detected?
Deepfake detection techniques typically involve analyzing visual or audio cues that deviate from natural human behavior. This can include examining inconsistencies in facial expressions, eye movements, lighting, skin tones, or unusual artifacts that may be present in the manipulated media. Some approaches involve using machine learning algorithms to distinguish deepfakes from authentic content.
Question 4: What countermeasures are being developed to detect deepfakes?
Researchers and technology companies are actively developing various countermeasures to detect deepfakes. These include improving deepfake detection algorithms, using authentication mechanisms, developing watermarking techniques, and promoting media literacy and education to raise awareness about the existence and potential dangers of deepfakes.
Question 5: Are there any tools available for users to detect deepfakes?
Yes, there are some tools and software available that claim to detect deepfakes. These tools may use different approaches such as analyzing facial landmarks, examining video compression artifacts, or utilizing artificial intelligence algorithms. However, it is important to note that deepfake technology is evolving rapidly, and the effectiveness of these tools may vary.
Question 6: Can deepfake detection be 100% accurate?
No, achieving 100% accuracy in deepfake detection is challenging. As deepfake technology advances, so do the techniques to create undetectable deepfakes. Moreover, some deepfake detection methods may produce false positives or false negatives, leading to potential misidentification or letting certain deepfakes slip through undetected.
Question 7: Can social media platforms detect and remove deepfake content?
Social media platforms are actively working on detecting and removing deepfake content. They employ various techniques, such as automated algorithms and human moderators, to identify and take down deepfakes that violate their content policies. However, it remains an ongoing challenge to detect and remove deepfakes at scale.
Question 8: How can individuals protect themselves against deepfakes?
Individuals can protect themselves against deepfakes by being cautious and critical consumers of media. It is important to verify the authenticity of media content before sharing or believing it. Users can also enable privacy settings on social media platforms, avoid sharing personal information online, and stay updated about deepfake detection techniques and awareness campaigns.
Question 9: Are there any laws or regulations against deepfakes?
Several countries have started creating laws and regulations to address the issues surrounding deepfakes. These laws often focus on issues such as defamation, privacy, copyright infringement, and the intent to deceive. However, the legal landscape is still evolving, and creating comprehensive legislation to combat deepfakes remains a complex task.
Question 10: Can deepfake technology be used for beneficial purposes?
While deepfake technology has raised concerns due to its potential for misuse, it can also be used for beneficial purposes. For example, it can be used in the entertainment industry for special effects or to resurrect deceased actors for film roles. Deepfakes can also be utilized in research, training, and educational contexts to simulate scenarios and improve understanding.